The Balancing Act of AI: Navigating Catastrophic Overtraining in Language Models
April 2, 2025, 4:36 am
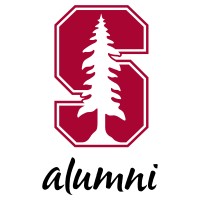
Location: United Kingdom, England, City of London
Employees: 10001+
Founded date: 1885
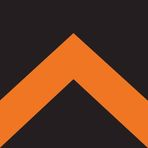
Location: United States, New Jersey, Princeton
Employees: 5001-10000
Founded date: 1746
In the fast-paced world of artificial intelligence, the race to develop larger and more capable language models (LLMs) is akin to a high-stakes game of poker. The stakes are high, and the players are betting on data. But a recent study reveals a critical flaw in this strategy: the phenomenon of "Catastrophic Overtraining." This term, coined by researchers from top institutions like Carnegie Mellon and Stanford, warns that more data isn't always better. Instead, it can lead to diminishing returns, making models less adaptable and effective.
Imagine a sponge. When you soak it in water, it absorbs and expands. But if you keep pouring water, it eventually overflows, losing its ability to hold more. Similarly, LLMs can become over-saturated with data, resulting in a decline in performance when fine-tuned for specific tasks. The study, titled "Overtrained Language Models Are Harder to Fine-Tune," highlights this critical inflection point in model training.
The researchers conducted a series of experiments comparing two versions of the AI2 OLMo-1B model. One was trained on 2.3 trillion tokens, while the other absorbed 3 trillion. Surprisingly, the latter performed worse after fine-tuning. This finding is not an isolated incident; it’s a consistent trend that raises alarms about the current trajectory of AI development.
At the heart of this issue lies "progressive sensitivity." As models undergo extensive pre-training, their parameters become increasingly sensitive to changes. This heightened fragility means that even minor adjustments during fine-tuning can lead to significant performance degradation. It’s like a tightrope walker who, after too much practice, becomes overly cautious and wobbly, losing the confidence to balance.
The researchers identified an inflection point around 2.5 trillion tokens. Beyond this threshold, additional training leads to diminishing returns. The implications are profound. For businesses and developers, this means that simply throwing more data at a model is not a guaranteed path to success. Instead, it can result in a fragile system that struggles to adapt to new tasks.
The study’s findings challenge the prevailing wisdom in AI development. Many believe that larger datasets equate to better models. However, this research suggests a more nuanced approach. It’s not just about quantity; quality and adaptability matter. Developers must weigh the benefits of extensive pre-training against the risks of catastrophic overtraining.
In practical terms, this means that organizations should consider fine-tuning smaller models trained on less data. The research indicates that these models may yield more reliable results in production environments. It’s a classic case of "less is more." The goal should be to optimize performance without succumbing to the pitfalls of overtraining.
The researchers also emphasize the need for further exploration into the factors influencing catastrophic overtraining. Questions remain about the role of pre-training optimizers, training objectives, and data distribution. Understanding these elements could lead to better strategies for model development.
As the AI landscape evolves, the implications of this research extend beyond technical considerations. They touch on ethical and practical aspects of AI deployment. If organizations prioritize data quantity over adaptability, they risk creating systems that are not only ineffective but also potentially harmful. A model that cannot adapt is like a ship without a rudder, drifting aimlessly.
In a world where AI is increasingly integrated into daily life, the stakes are high. Businesses rely on LLMs for everything from customer service to content creation. If these models are prone to catastrophic overtraining, the consequences could be far-reaching. Organizations must navigate this landscape with caution, balancing the desire for powerful models with the need for reliable performance.
The study serves as a wake-up call for the AI community. It underscores the importance of a balanced approach to model training. Developers must prioritize adaptability and performance over sheer data volume. This shift in mindset could lead to more robust and effective AI systems.
In conclusion, the phenomenon of catastrophic overtraining is a critical consideration for anyone involved in AI development. As researchers continue to explore this complex issue, the lessons learned will shape the future of language models. The path forward requires a delicate balance—a dance between data and adaptability. In this high-stakes game, understanding when to hold back could be the key to success.
Imagine a sponge. When you soak it in water, it absorbs and expands. But if you keep pouring water, it eventually overflows, losing its ability to hold more. Similarly, LLMs can become over-saturated with data, resulting in a decline in performance when fine-tuned for specific tasks. The study, titled "Overtrained Language Models Are Harder to Fine-Tune," highlights this critical inflection point in model training.
The researchers conducted a series of experiments comparing two versions of the AI2 OLMo-1B model. One was trained on 2.3 trillion tokens, while the other absorbed 3 trillion. Surprisingly, the latter performed worse after fine-tuning. This finding is not an isolated incident; it’s a consistent trend that raises alarms about the current trajectory of AI development.
At the heart of this issue lies "progressive sensitivity." As models undergo extensive pre-training, their parameters become increasingly sensitive to changes. This heightened fragility means that even minor adjustments during fine-tuning can lead to significant performance degradation. It’s like a tightrope walker who, after too much practice, becomes overly cautious and wobbly, losing the confidence to balance.
The researchers identified an inflection point around 2.5 trillion tokens. Beyond this threshold, additional training leads to diminishing returns. The implications are profound. For businesses and developers, this means that simply throwing more data at a model is not a guaranteed path to success. Instead, it can result in a fragile system that struggles to adapt to new tasks.
The study’s findings challenge the prevailing wisdom in AI development. Many believe that larger datasets equate to better models. However, this research suggests a more nuanced approach. It’s not just about quantity; quality and adaptability matter. Developers must weigh the benefits of extensive pre-training against the risks of catastrophic overtraining.
In practical terms, this means that organizations should consider fine-tuning smaller models trained on less data. The research indicates that these models may yield more reliable results in production environments. It’s a classic case of "less is more." The goal should be to optimize performance without succumbing to the pitfalls of overtraining.
The researchers also emphasize the need for further exploration into the factors influencing catastrophic overtraining. Questions remain about the role of pre-training optimizers, training objectives, and data distribution. Understanding these elements could lead to better strategies for model development.
As the AI landscape evolves, the implications of this research extend beyond technical considerations. They touch on ethical and practical aspects of AI deployment. If organizations prioritize data quantity over adaptability, they risk creating systems that are not only ineffective but also potentially harmful. A model that cannot adapt is like a ship without a rudder, drifting aimlessly.
In a world where AI is increasingly integrated into daily life, the stakes are high. Businesses rely on LLMs for everything from customer service to content creation. If these models are prone to catastrophic overtraining, the consequences could be far-reaching. Organizations must navigate this landscape with caution, balancing the desire for powerful models with the need for reliable performance.
The study serves as a wake-up call for the AI community. It underscores the importance of a balanced approach to model training. Developers must prioritize adaptability and performance over sheer data volume. This shift in mindset could lead to more robust and effective AI systems.
In conclusion, the phenomenon of catastrophic overtraining is a critical consideration for anyone involved in AI development. As researchers continue to explore this complex issue, the lessons learned will shape the future of language models. The path forward requires a delicate balance—a dance between data and adaptability. In this high-stakes game, understanding when to hold back could be the key to success.