Composo: Navigating the Uncertainty of AI Application Performance
February 8, 2025, 4:07 am
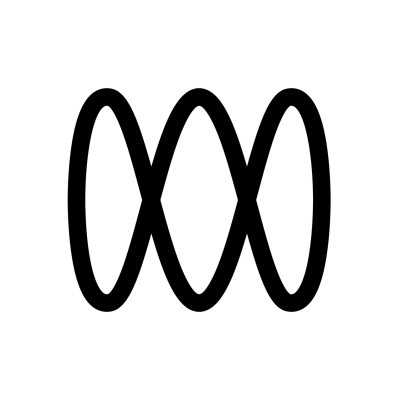
Location: United Kingdom, England, London
Employees: 1-10
Founded date: 2020
Total raised: $2.6M
In the fast-paced world of artificial intelligence (AI), uncertainty looms large. Companies invest heavily in AI applications, yet reliability remains a distant dream. Enter Composo, a London-based startup that aims to bridge this gap. With its innovative approach, Composo is poised to help businesses assess the performance of AI applications powered by large language models (LLMs).
AI is a double-edged sword. It promises efficiency and insight but often falls short on reliability. Businesses are left wondering if their investments yield the expected results. Composo sees this uncertainty as an opportunity. The startup has developed unique models that allow enterprises to evaluate the accuracy and quality of their AI applications.
Composo is not alone in this quest. It joins the ranks of other startups like Agenta, Freeplay, Humanloop, and LangSmith. Each claims to offer a more reliable alternative to traditional human testing and existing monitoring tools. However, Composo sets itself apart by providing both a no-code option and an API. This flexibility opens the door for a broader audience. You don’t need to be a developer to use Composo. Subject matter experts and managers can independently assess AI applications for discrepancies, quality, and accuracy.
At its core, Composo combines a reward model trained on preferred outcomes with specific criteria tailored to each application. This system evaluates the performance of AI applications against these criteria. For instance, a medical chatbot can establish guidelines for identifying concerning symptoms. Composo then measures how consistently the application adheres to these guidelines.
Recently, Composo launched a public API for Composo Align, a model designed to evaluate LLM applications based on any criteria. This move is a significant step forward, enabling businesses to customize their assessments. The strategy appears to be paying off. Composo has attracted notable clients, including Accenture, Palantir, and McKinsey. The startup recently secured $2 million in seed funding, a modest sum in the AI landscape but indicative of its potential.
Sebastian Fox, co-founder and CEO of Composo, explains that the relatively small funding amount is due to the startup's low capital requirements. Unlike many competitors that require massive investments, Composo's approach is efficient. The focus is not on building foundational models but on enhancing existing applications. This strategy allows Composo to thrive even as larger players make headlines with their breakthroughs.
With the new funding, Composo plans to expand its engineering team, led by co-founder and CTO Luke Markham, a former machine learning engineer at Graphcore. The goal is to attract more clients and bolster research and development efforts. The company aims to scale its existing technologies, enhancing its offerings for current clients.
Twin Path Ventures, a UK-based seed fund specializing in AI, led the funding round, joined by JVH Ventures and EWOR. The backing from these investors underscores the belief in Composo's potential to eliminate critical bottlenecks in corporate AI adoption. Fox emphasizes that this bottleneck is a significant hurdle for the entire AI movement, particularly in the corporate sector. Businesses are now more cautious, questioning whether AI truly transforms their operations. They seek assurance of reliability and consistency.
Composo's value proposition lies in its ability to provide that assurance. The startup positions itself as industry-agnostic while resonating in sectors like compliance, law, healthcare, and security. This broad applicability enhances its appeal to companies wary of reputational risks associated with unreliable AI.
When it comes to competitive advantage, Fox asserts that the research and development required to achieve Composo's goals are non-trivial. The architecture of the model and the data used for training are crucial. Composo Align has been trained on a vast dataset of expert evaluations, setting it apart from competitors.
The question remains: what could tech giants achieve if they applied their vast resources to this problem? Composo believes it has a first-mover advantage. The data it accumulates over time enhances its evaluation preferences, creating a unique position in the market.
As Composo evaluates applications using a flexible set of criteria, it believes it is better equipped to develop agent-based AI than competitors with a more limited approach. Fox notes that the current state of agent-based AI is far from optimal, and Composo aims to address this challenge.
In conclusion, Composo stands at the intersection of opportunity and uncertainty. The startup is not just another player in the AI landscape; it is a beacon for businesses seeking reliability in their AI applications. As it continues to refine its models and expand its reach, Composo may very well redefine how companies assess and implement AI technology. The journey is just beginning, and the potential is vast.
AI is a double-edged sword. It promises efficiency and insight but often falls short on reliability. Businesses are left wondering if their investments yield the expected results. Composo sees this uncertainty as an opportunity. The startup has developed unique models that allow enterprises to evaluate the accuracy and quality of their AI applications.
Composo is not alone in this quest. It joins the ranks of other startups like Agenta, Freeplay, Humanloop, and LangSmith. Each claims to offer a more reliable alternative to traditional human testing and existing monitoring tools. However, Composo sets itself apart by providing both a no-code option and an API. This flexibility opens the door for a broader audience. You don’t need to be a developer to use Composo. Subject matter experts and managers can independently assess AI applications for discrepancies, quality, and accuracy.
At its core, Composo combines a reward model trained on preferred outcomes with specific criteria tailored to each application. This system evaluates the performance of AI applications against these criteria. For instance, a medical chatbot can establish guidelines for identifying concerning symptoms. Composo then measures how consistently the application adheres to these guidelines.
Recently, Composo launched a public API for Composo Align, a model designed to evaluate LLM applications based on any criteria. This move is a significant step forward, enabling businesses to customize their assessments. The strategy appears to be paying off. Composo has attracted notable clients, including Accenture, Palantir, and McKinsey. The startup recently secured $2 million in seed funding, a modest sum in the AI landscape but indicative of its potential.
Sebastian Fox, co-founder and CEO of Composo, explains that the relatively small funding amount is due to the startup's low capital requirements. Unlike many competitors that require massive investments, Composo's approach is efficient. The focus is not on building foundational models but on enhancing existing applications. This strategy allows Composo to thrive even as larger players make headlines with their breakthroughs.
With the new funding, Composo plans to expand its engineering team, led by co-founder and CTO Luke Markham, a former machine learning engineer at Graphcore. The goal is to attract more clients and bolster research and development efforts. The company aims to scale its existing technologies, enhancing its offerings for current clients.
Twin Path Ventures, a UK-based seed fund specializing in AI, led the funding round, joined by JVH Ventures and EWOR. The backing from these investors underscores the belief in Composo's potential to eliminate critical bottlenecks in corporate AI adoption. Fox emphasizes that this bottleneck is a significant hurdle for the entire AI movement, particularly in the corporate sector. Businesses are now more cautious, questioning whether AI truly transforms their operations. They seek assurance of reliability and consistency.
Composo's value proposition lies in its ability to provide that assurance. The startup positions itself as industry-agnostic while resonating in sectors like compliance, law, healthcare, and security. This broad applicability enhances its appeal to companies wary of reputational risks associated with unreliable AI.
When it comes to competitive advantage, Fox asserts that the research and development required to achieve Composo's goals are non-trivial. The architecture of the model and the data used for training are crucial. Composo Align has been trained on a vast dataset of expert evaluations, setting it apart from competitors.
The question remains: what could tech giants achieve if they applied their vast resources to this problem? Composo believes it has a first-mover advantage. The data it accumulates over time enhances its evaluation preferences, creating a unique position in the market.
As Composo evaluates applications using a flexible set of criteria, it believes it is better equipped to develop agent-based AI than competitors with a more limited approach. Fox notes that the current state of agent-based AI is far from optimal, and Composo aims to address this challenge.
In conclusion, Composo stands at the intersection of opportunity and uncertainty. The startup is not just another player in the AI landscape; it is a beacon for businesses seeking reliability in their AI applications. As it continues to refine its models and expand its reach, Composo may very well redefine how companies assess and implement AI technology. The journey is just beginning, and the potential is vast.