The Rise of Open-Source AI: Alibaba's Qwen Models and the Future of Data Privacy
January 30, 2025, 3:39 am
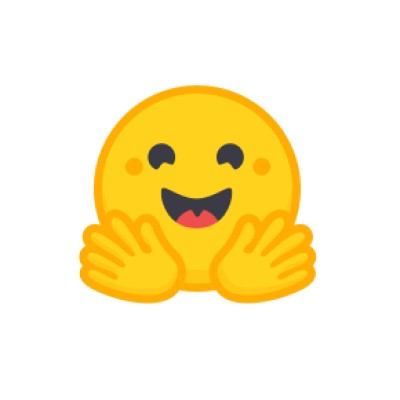
Location: Australia, New South Wales, Concord
Employees: 51-200
Founded date: 2016
Total raised: $494M
In the world of artificial intelligence, innovation is the lifeblood. Recently, Alibaba unveiled its Qwen models, a significant leap in open-source AI technology. These models, Qwen2.5-7B-Instruct-1M and Qwen2.5-14B-Instruct-1M, can process a staggering one million tokens. This capability sets them apart in a crowded field. Imagine a librarian who can read an entire library in seconds, picking out only the most crucial information. That’s the essence of Qwen.
The architecture of these models employs a unique attention mechanism. Instead of drowning in data, they focus on the vital parts of the context. This selective attention allows them to operate three to seven times faster than traditional models. They can churn out responses containing up to 8,000 tokens. However, the challenge remains: identifying key excerpts from lengthy documents. Many existing models falter here, like a student struggling to summarize a dense textbook.
Testing has shown that both the 14B and 7B models excel at extracting hidden numbers from vast text arrays. The 14B model even scored over 90 in the RULER test, a first for the Qwen series. This performance is akin to a sprinter breaking a record, leaving competitors in the dust. Yet, these tests primarily assess information retrieval, not deep comprehension. It’s like checking if a student can find answers but not if they truly understand the material.
The advantages of large context windows are not always clear-cut. While they offer flexibility, Retrieval-Augmented Generation (RAG) systems often outperform them with smaller context windows. RAG systems pull information from external databases during inference, proving more accurate and efficient. However, in complex evaluations like RULER and LongbenchChat, the Qwen models shine, especially with sequences longer than 64,000 tokens.
For users eager to explore these models, Alibaba provides access through Qwen Chat, a platform reminiscent of ChatGPT. This opens the door for developers and businesses to harness the power of Qwen without hefty investments. In a landscape dominated by American tech giants, Alibaba’s offerings present a competitive edge, especially at lower costs.
Meanwhile, the conversation around data privacy continues to evolve. The Russian initiative, dubbed "Russian Masquerade," focuses on protecting personal data through Named Entity Recognition (NER). This technology identifies and masks sensitive information in texts. Picture a magician pulling a rabbit out of a hat, but instead, they’re pulling personal details out of documents and hiding them from view.
The global landscape of data protection is complex. Laws vary significantly across regions, from Russia’s 152-FZ to the EU’s General Data Protection Regulation. This regulatory maze can feel like navigating a labyrinth. The goal is clear: safeguard personal data while leveraging technological advancements. NER plays a crucial role in this endeavor, allowing organizations to process data without compromising privacy.
The "Russian Masquerade" team utilized a dataset of 1,150 phrases to evaluate their NER models. They aimed to measure Recall, the ability to identify named entities accurately. Think of Recall as a detective’s success rate in solving cases. A high Recall means the detective catches most criminals, while a low Recall indicates many slip through the cracks.
Among the models tested, Slavic BERT emerged as a strong contender. This multilingual model, adapted for Slavic languages, demonstrated a Recall of 76.4%. While this is commendable, it still leaves room for improvement. The quest for better accuracy led the team to explore other models, such as GLiNER and Spacy.
GLiNER, a compact model, showed impressive results with a Recall of 94.9%. It’s like finding a reliable tool that fits perfectly in your toolbox. Meanwhile, Spacy, a popular NLP library, achieved a Recall of 95.4% through a combination of real and synthetic data. This iterative approach allowed for greater versatility in entity extraction.
The Qwen models also entered the fray, with the Qwen2.5-Coder-7B-Instruct-AWQ achieving a Recall of 87.4%. While not the highest, it showcased the potential of integrating advanced AI into data privacy solutions. The team experimented with combinations of models, ultimately achieving a remarkable Recall of 98.6% by merging GLiNER and Qwen.
This collaboration illustrates the power of synergy in technology. Just as a well-orchestrated symphony produces beautiful music, combining different models can yield superior results. The landscape of AI and data privacy is rapidly changing. With advancements like Alibaba’s Qwen models and innovative approaches to NER, the future looks promising.
As we navigate this evolving terrain, the balance between innovation and privacy remains crucial. The ability to harness powerful AI while protecting personal data is not just a goal; it’s a necessity. The stakes are high, and the path forward is filled with opportunities. The rise of open-source AI is just the beginning. The journey ahead promises to be as thrilling as it is challenging.
The architecture of these models employs a unique attention mechanism. Instead of drowning in data, they focus on the vital parts of the context. This selective attention allows them to operate three to seven times faster than traditional models. They can churn out responses containing up to 8,000 tokens. However, the challenge remains: identifying key excerpts from lengthy documents. Many existing models falter here, like a student struggling to summarize a dense textbook.
Testing has shown that both the 14B and 7B models excel at extracting hidden numbers from vast text arrays. The 14B model even scored over 90 in the RULER test, a first for the Qwen series. This performance is akin to a sprinter breaking a record, leaving competitors in the dust. Yet, these tests primarily assess information retrieval, not deep comprehension. It’s like checking if a student can find answers but not if they truly understand the material.
The advantages of large context windows are not always clear-cut. While they offer flexibility, Retrieval-Augmented Generation (RAG) systems often outperform them with smaller context windows. RAG systems pull information from external databases during inference, proving more accurate and efficient. However, in complex evaluations like RULER and LongbenchChat, the Qwen models shine, especially with sequences longer than 64,000 tokens.
For users eager to explore these models, Alibaba provides access through Qwen Chat, a platform reminiscent of ChatGPT. This opens the door for developers and businesses to harness the power of Qwen without hefty investments. In a landscape dominated by American tech giants, Alibaba’s offerings present a competitive edge, especially at lower costs.
Meanwhile, the conversation around data privacy continues to evolve. The Russian initiative, dubbed "Russian Masquerade," focuses on protecting personal data through Named Entity Recognition (NER). This technology identifies and masks sensitive information in texts. Picture a magician pulling a rabbit out of a hat, but instead, they’re pulling personal details out of documents and hiding them from view.
The global landscape of data protection is complex. Laws vary significantly across regions, from Russia’s 152-FZ to the EU’s General Data Protection Regulation. This regulatory maze can feel like navigating a labyrinth. The goal is clear: safeguard personal data while leveraging technological advancements. NER plays a crucial role in this endeavor, allowing organizations to process data without compromising privacy.
The "Russian Masquerade" team utilized a dataset of 1,150 phrases to evaluate their NER models. They aimed to measure Recall, the ability to identify named entities accurately. Think of Recall as a detective’s success rate in solving cases. A high Recall means the detective catches most criminals, while a low Recall indicates many slip through the cracks.
Among the models tested, Slavic BERT emerged as a strong contender. This multilingual model, adapted for Slavic languages, demonstrated a Recall of 76.4%. While this is commendable, it still leaves room for improvement. The quest for better accuracy led the team to explore other models, such as GLiNER and Spacy.
GLiNER, a compact model, showed impressive results with a Recall of 94.9%. It’s like finding a reliable tool that fits perfectly in your toolbox. Meanwhile, Spacy, a popular NLP library, achieved a Recall of 95.4% through a combination of real and synthetic data. This iterative approach allowed for greater versatility in entity extraction.
The Qwen models also entered the fray, with the Qwen2.5-Coder-7B-Instruct-AWQ achieving a Recall of 87.4%. While not the highest, it showcased the potential of integrating advanced AI into data privacy solutions. The team experimented with combinations of models, ultimately achieving a remarkable Recall of 98.6% by merging GLiNER and Qwen.
This collaboration illustrates the power of synergy in technology. Just as a well-orchestrated symphony produces beautiful music, combining different models can yield superior results. The landscape of AI and data privacy is rapidly changing. With advancements like Alibaba’s Qwen models and innovative approaches to NER, the future looks promising.
As we navigate this evolving terrain, the balance between innovation and privacy remains crucial. The ability to harness powerful AI while protecting personal data is not just a goal; it’s a necessity. The stakes are high, and the path forward is filled with opportunities. The rise of open-source AI is just the beginning. The journey ahead promises to be as thrilling as it is challenging.