The Evolving Landscape of AI Text Detection and Look-a-Like Pseudolabeling
January 29, 2025, 5:15 pm
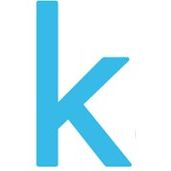
Location: United States, California, San Francisco
Employees: 11-50
Founded date: 2009
Total raised: $11M
In the world of artificial intelligence, the line between human and machine-generated text is blurring. As AI models grow more sophisticated, the challenge of distinguishing between the two becomes a game of cat and mouse. This article explores the intricacies of AI text detection and the innovative method of look-a-like pseudolabeling in marketing.
The journey begins with the rise of large language models (LLMs). These models, like GPT-4 and others, have advanced to a point where their outputs can mimic human writing styles with alarming accuracy. The question looms: Can we still tell the difference between AI-generated text and that crafted by a human hand?
The quest for answers started in competitions like Kaggle's "LLM - Detect AI Generated Text." Participants employed various strategies to classify text. They analyzed patterns, looking for telltale signs of AI involvement. Repeated phrases, monotonous tones, and overly formal language became red flags. Yet, as models improved, these indicators became less reliable.
AI text detection tools emerged, each claiming to unravel the mystery. GPTZero, for instance, gained popularity as a teacher's ally, assessing the likelihood of AI authorship. But the landscape is ever-changing. New models are released, and detection tools struggle to keep pace. The result? A relentless arms race between creators and detectors.
The situation is further complicated by the emergence of tools designed to obfuscate AI-generated text. Services like Undetectable AI aim to reformat content, making it undetectable by existing tools. This dynamic creates a perpetual cycle of innovation and counter-innovation.
Despite the challenges, there are still ways to identify AI-generated text. Less advanced models often leave traces of their artificiality. Patterns of repetition and lack of depth can signal machine authorship. However, when humans edit AI outputs, the task becomes significantly harder. The question remains: Is there a foolproof method for detection?
As we navigate this complex terrain, the focus shifts to practical applications. One such application is in marketing, where businesses seek to expand their customer base. Enter look-a-like pseudolabeling, a technique that leverages existing customer data to identify potential new clients.
Imagine a business owner with a modest clientele of 200-300 customers. They want to scale up, targeting thousands more. The challenge lies in finding individuals who resemble their current customers. This is where data science steps in.
Using a dataset of potential clients, data scientists can create a model to predict which individuals are most likely to convert. By employing a binary classification approach, they can sift through the data, identifying those who share characteristics with existing customers. The goal is to maximize the chances of successful outreach.
In this scenario, the process begins with data generation. A synthetic dataset is created, simulating potential customers with various features. From this pool, a subset of known customers is selected, while others remain unlabeled. The task is to identify the hidden gems among the unlabeled data.
The first step involves training a model on the labeled data. This model learns to recognize patterns associated with existing customers. Once trained, it can make predictions on the unlabeled data, estimating the likelihood of each individual being a potential customer.
However, the initial model's predictions are not the end of the story. To enhance accuracy, data scientists can employ pseudolabeling. This technique involves using the model's predictions to create new labels for the unlabeled data. By iterating through the process, the model becomes more refined, improving its ability to identify potential customers.
The results can be staggering. A traditional approach might yield a mere 2.3% success rate in identifying potential customers. A basic model could improve this to 15.4%. But with pseudolabeling, the success rate can soar to 80%. This leap demonstrates the power of combining machine learning techniques with domain knowledge.
As businesses strive to optimize their marketing efforts, the integration of AI and data science becomes paramount. The ability to identify look-a-like customers not only enhances outreach but also maximizes return on investment. In a world where every dollar counts, this approach can be a game-changer.
In conclusion, the landscape of AI text detection and marketing strategies is evolving rapidly. The challenge of distinguishing between human and machine-generated text persists, but innovative solutions continue to emerge. Look-a-like pseudolabeling stands as a testament to the potential of data science in driving business success. As we move forward, the interplay between AI and human creativity will shape the future of communication and marketing. The race is on, and those who adapt will thrive.
The journey begins with the rise of large language models (LLMs). These models, like GPT-4 and others, have advanced to a point where their outputs can mimic human writing styles with alarming accuracy. The question looms: Can we still tell the difference between AI-generated text and that crafted by a human hand?
The quest for answers started in competitions like Kaggle's "LLM - Detect AI Generated Text." Participants employed various strategies to classify text. They analyzed patterns, looking for telltale signs of AI involvement. Repeated phrases, monotonous tones, and overly formal language became red flags. Yet, as models improved, these indicators became less reliable.
AI text detection tools emerged, each claiming to unravel the mystery. GPTZero, for instance, gained popularity as a teacher's ally, assessing the likelihood of AI authorship. But the landscape is ever-changing. New models are released, and detection tools struggle to keep pace. The result? A relentless arms race between creators and detectors.
The situation is further complicated by the emergence of tools designed to obfuscate AI-generated text. Services like Undetectable AI aim to reformat content, making it undetectable by existing tools. This dynamic creates a perpetual cycle of innovation and counter-innovation.
Despite the challenges, there are still ways to identify AI-generated text. Less advanced models often leave traces of their artificiality. Patterns of repetition and lack of depth can signal machine authorship. However, when humans edit AI outputs, the task becomes significantly harder. The question remains: Is there a foolproof method for detection?
As we navigate this complex terrain, the focus shifts to practical applications. One such application is in marketing, where businesses seek to expand their customer base. Enter look-a-like pseudolabeling, a technique that leverages existing customer data to identify potential new clients.
Imagine a business owner with a modest clientele of 200-300 customers. They want to scale up, targeting thousands more. The challenge lies in finding individuals who resemble their current customers. This is where data science steps in.
Using a dataset of potential clients, data scientists can create a model to predict which individuals are most likely to convert. By employing a binary classification approach, they can sift through the data, identifying those who share characteristics with existing customers. The goal is to maximize the chances of successful outreach.
In this scenario, the process begins with data generation. A synthetic dataset is created, simulating potential customers with various features. From this pool, a subset of known customers is selected, while others remain unlabeled. The task is to identify the hidden gems among the unlabeled data.
The first step involves training a model on the labeled data. This model learns to recognize patterns associated with existing customers. Once trained, it can make predictions on the unlabeled data, estimating the likelihood of each individual being a potential customer.
However, the initial model's predictions are not the end of the story. To enhance accuracy, data scientists can employ pseudolabeling. This technique involves using the model's predictions to create new labels for the unlabeled data. By iterating through the process, the model becomes more refined, improving its ability to identify potential customers.
The results can be staggering. A traditional approach might yield a mere 2.3% success rate in identifying potential customers. A basic model could improve this to 15.4%. But with pseudolabeling, the success rate can soar to 80%. This leap demonstrates the power of combining machine learning techniques with domain knowledge.
As businesses strive to optimize their marketing efforts, the integration of AI and data science becomes paramount. The ability to identify look-a-like customers not only enhances outreach but also maximizes return on investment. In a world where every dollar counts, this approach can be a game-changer.
In conclusion, the landscape of AI text detection and marketing strategies is evolving rapidly. The challenge of distinguishing between human and machine-generated text persists, but innovative solutions continue to emerge. Look-a-like pseudolabeling stands as a testament to the potential of data science in driving business success. As we move forward, the interplay between AI and human creativity will shape the future of communication and marketing. The race is on, and those who adapt will thrive.