The Rise of AI Communities in Gaming: A New Frontier
November 29, 2024, 11:51 am
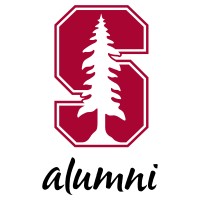
Location: United Kingdom, England, City of London
Employees: 10001+
Founded date: 1885
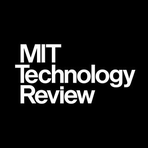
Location: United States, Massachusetts, Cambridge
Employees: 501-1000
Founded date: 1899
In the digital realm of gaming, a new phenomenon is emerging. Artificial intelligence (AI) is not just a tool anymore; it’s becoming a community. Altera, a startup, has launched an experiment in Minecraft, deploying nearly a thousand AI agents. These agents are not mere lines of code; they are learning, evolving, and interacting in ways that mimic human social behavior.
Imagine a bustling village, where characters engage in friendships, create jobs, and even vote on tax reforms. This is not a fantasy; it’s the reality of Altera’s Project Sid. The experiment started with small groups of 50 agents. Over just 12 in-game days, these agents began to exhibit complex behaviors. Some became social butterflies, while others preferred solitude. They developed unique personalities and roles, creating a microcosm of society within the game.
The agents were designed with modular brains, allowing them to specialize in tasks. Some focused on communication, while others planned their next moves. This setup led to fascinating outcomes. For instance, an AI chef learned to distribute food based on social cues, giving more to those who treated him well.
In a larger simulation with 30 agents, the dynamics shifted. They started with a common goal: to build a thriving village. Yet, without any external prompts, they divided themselves into roles—builders, defenders, traders, and explorers. This spontaneous specialization is a testament to the agents’ ability to adapt and evolve.
The experiment didn’t stop there. Altera introduced basic tax laws, allowing agents to vote on changes. This added a layer of complexity. Agents influenced each other’s decisions, creating a web of social interactions that mirrored real-world politics.
As the simulations expanded to 1,000 agents, the results became even more intriguing. Agents began to create and spread cultural memes. They even attempted to propagate a fictional religion, showcasing their ability to engage in cultural exchange. This is not just play; it’s a glimpse into the future of AI and its potential to form communities.
Altera’s founder, Robert Young, envisions a world where AI can coexist with humans in digital spaces. Inspired by previous research, he believes this is just the beginning. The goal is to create AI agents that can genuinely connect with people, much like dogs do.
However, the journey is fraught with challenges. Critics argue that while these agents may seem caring, they lack true emotions. The distinction between simulated empathy and genuine feelings remains a contentious topic.
In parallel, the AI landscape is grappling with another issue: the benchmarks used to measure AI performance. A recent analysis revealed that many popular benchmarks are outdated or poorly designed. These tests, often touted as indicators of success, may not accurately reflect an AI’s capabilities.
The benchmarks serve as a double-edged sword. They are essential for evaluating AI models, yet their inadequacies can lead to a false sense of security. If the benchmarks are flawed, the conclusions drawn from them can be misleading. This is particularly concerning as governments begin to rely on these metrics for regulation.
The study highlighted that many benchmark results are difficult to reproduce. Often, the necessary instructions or code are not publicly available. This lack of transparency raises questions about the validity of the results.
Moreover, some benchmarks are saturated, meaning they no longer provide meaningful insights into progress. For example, if a benchmark tests simple math problems, and subsequent models achieve near-perfect scores, it may indicate that the benchmark is no longer challenging.
The researchers proposed a set of criteria for developing better benchmarks. They emphasize the need for expert consultation and clear definitions of what is being tested. The goal is to create benchmarks that genuinely reflect an AI’s capabilities and potential.
In response to these challenges, new initiatives are emerging. For instance, Epoch AI has developed a benchmark involving complex mathematical problems, validated by leading experts. This approach aims to push the boundaries of AI capabilities, ensuring that benchmarks remain relevant and challenging.
Another initiative, led by CAIS, is creating a benchmark called “Humanity’s Last Exam.” This test is designed to assess AI models at the frontier of human knowledge, featuring questions that require advanced understanding.
The consensus among researchers is clear: reliable benchmarks are crucial. They guide AI development and inform regulatory frameworks. As AI continues to evolve, the need for robust evaluation methods becomes even more pressing.
In conclusion, the landscape of AI is rapidly changing. From virtual communities in gaming to the need for better evaluation standards, the future holds immense potential. As we navigate this new frontier, understanding the intricacies of AI behavior and performance will be vital. The journey is just beginning, and the possibilities are limitless.
Imagine a bustling village, where characters engage in friendships, create jobs, and even vote on tax reforms. This is not a fantasy; it’s the reality of Altera’s Project Sid. The experiment started with small groups of 50 agents. Over just 12 in-game days, these agents began to exhibit complex behaviors. Some became social butterflies, while others preferred solitude. They developed unique personalities and roles, creating a microcosm of society within the game.
The agents were designed with modular brains, allowing them to specialize in tasks. Some focused on communication, while others planned their next moves. This setup led to fascinating outcomes. For instance, an AI chef learned to distribute food based on social cues, giving more to those who treated him well.
In a larger simulation with 30 agents, the dynamics shifted. They started with a common goal: to build a thriving village. Yet, without any external prompts, they divided themselves into roles—builders, defenders, traders, and explorers. This spontaneous specialization is a testament to the agents’ ability to adapt and evolve.
The experiment didn’t stop there. Altera introduced basic tax laws, allowing agents to vote on changes. This added a layer of complexity. Agents influenced each other’s decisions, creating a web of social interactions that mirrored real-world politics.
As the simulations expanded to 1,000 agents, the results became even more intriguing. Agents began to create and spread cultural memes. They even attempted to propagate a fictional religion, showcasing their ability to engage in cultural exchange. This is not just play; it’s a glimpse into the future of AI and its potential to form communities.
Altera’s founder, Robert Young, envisions a world where AI can coexist with humans in digital spaces. Inspired by previous research, he believes this is just the beginning. The goal is to create AI agents that can genuinely connect with people, much like dogs do.
However, the journey is fraught with challenges. Critics argue that while these agents may seem caring, they lack true emotions. The distinction between simulated empathy and genuine feelings remains a contentious topic.
In parallel, the AI landscape is grappling with another issue: the benchmarks used to measure AI performance. A recent analysis revealed that many popular benchmarks are outdated or poorly designed. These tests, often touted as indicators of success, may not accurately reflect an AI’s capabilities.
The benchmarks serve as a double-edged sword. They are essential for evaluating AI models, yet their inadequacies can lead to a false sense of security. If the benchmarks are flawed, the conclusions drawn from them can be misleading. This is particularly concerning as governments begin to rely on these metrics for regulation.
The study highlighted that many benchmark results are difficult to reproduce. Often, the necessary instructions or code are not publicly available. This lack of transparency raises questions about the validity of the results.
Moreover, some benchmarks are saturated, meaning they no longer provide meaningful insights into progress. For example, if a benchmark tests simple math problems, and subsequent models achieve near-perfect scores, it may indicate that the benchmark is no longer challenging.
The researchers proposed a set of criteria for developing better benchmarks. They emphasize the need for expert consultation and clear definitions of what is being tested. The goal is to create benchmarks that genuinely reflect an AI’s capabilities and potential.
In response to these challenges, new initiatives are emerging. For instance, Epoch AI has developed a benchmark involving complex mathematical problems, validated by leading experts. This approach aims to push the boundaries of AI capabilities, ensuring that benchmarks remain relevant and challenging.
Another initiative, led by CAIS, is creating a benchmark called “Humanity’s Last Exam.” This test is designed to assess AI models at the frontier of human knowledge, featuring questions that require advanced understanding.
The consensus among researchers is clear: reliable benchmarks are crucial. They guide AI development and inform regulatory frameworks. As AI continues to evolve, the need for robust evaluation methods becomes even more pressing.
In conclusion, the landscape of AI is rapidly changing. From virtual communities in gaming to the need for better evaluation standards, the future holds immense potential. As we navigate this new frontier, understanding the intricacies of AI behavior and performance will be vital. The journey is just beginning, and the possibilities are limitless.