OpenScholar: A New Dawn for Scientific Research
November 24, 2024, 7:06 am
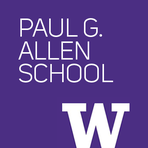
Location: United States, Washington, Seattle
Employees: 10001+
Founded date: 1861
Total raised: $800K
In the vast ocean of scientific literature, researchers often find themselves adrift. Millions of papers flood the market each year, making it nearly impossible to stay afloat. Enter OpenScholar, a revolutionary open-source AI designed to be the lifeboat in this stormy sea of data. Developed by the Allen Institute for AI and the University of Washington, OpenScholar is not just another AI tool; it’s a game-changer for how researchers access, evaluate, and synthesize scientific knowledge.
Imagine a world where researchers can navigate through 45 million academic papers in mere seconds. OpenScholar’s retrieval-augmented language model does just that. It doesn’t simply regurgitate pre-trained knowledge like its proprietary counterparts, such as GPT-4o. Instead, it actively retrieves relevant studies, synthesizes their findings, and delivers answers grounded in real literature. This ability to stay anchored in verifiable sources sets OpenScholar apart.
In a recent benchmark test called ScholarQABench, OpenScholar outperformed larger models like GPT-4o in factuality and citation accuracy. While GPT-4o often fabricates citations—over 90% of the time in biomedical queries—OpenScholar remains steadfast, relying on legitimate sources. This reliability is crucial in a field where accuracy can mean the difference between life and death.
OpenScholar’s self-feedback inference loop iteratively refines its outputs, enhancing quality and incorporating new information. This dynamic process allows researchers to receive accurate, citation-backed answers to complex questions. The implications are profound. OpenScholar could accelerate scientific discovery, enabling experts to synthesize knowledge faster and with greater confidence.
But OpenScholar isn’t just about performance; it’s about accessibility. In a landscape dominated by expensive, closed systems, OpenScholar flips the script. It’s fully open-source, offering not just the code but the entire retrieval pipeline. This democratization of technology means smaller institutions and underfunded labs can access powerful AI tools without breaking the bank. The researchers estimate that OpenScholar is 100 times cheaper to operate than its proprietary counterparts.
However, OpenScholar is not without its limitations. Its reliance on open-access papers means it may miss critical findings locked behind paywalls. This gap is particularly concerning in high-stakes fields like medicine, where much research remains inaccessible. The developers acknowledge this shortcoming and hope future iterations can responsibly incorporate closed-access content.
Despite these challenges, OpenScholar represents a significant shift in scientific computing. It’s not just about engaging in conversation; it’s about processing, understanding, and synthesizing scientific literature with near-human accuracy. The numbers speak volumes. OpenScholar’s 8-billion-parameter model not only outperforms GPT-4o but also matches human experts in citation accuracy, where other AIs fail dramatically.
The emergence of OpenScholar raises important questions about the role of AI in science. While it excels in synthesizing literature, it’s not infallible. In expert evaluations, OpenScholar’s answers were preferred over human-written responses 70% of the time. The remaining 30% highlighted areas for improvement, such as failing to cite foundational papers. This underscores a vital truth: AI tools are meant to augment, not replace, human expertise.
Critics may argue that OpenScholar’s limitations hinder its utility in certain fields. However, its strengths lie in its ability to assist researchers in handling the time-consuming task of literature synthesis. This allows scientists to focus on interpretation and advancing knowledge rather than getting lost in the data deluge.
OpenScholar’s debut comes at a crucial time. The AI ecosystem is increasingly dominated by proprietary systems that are expensive and opaque. OpenScholar’s open-source model not only challenges this status quo but also provides a pathway for innovation. By releasing everything from code to training recipes, the developers are betting that openness will accelerate progress more than keeping breakthroughs behind closed doors.
As we stand on the brink of a new era in AI-assisted research, the bottleneck in scientific progress may no longer be our ability to process existing knowledge. Instead, it may hinge on our capacity to ask the right questions. OpenScholar is a testament to this shift, demonstrating that open-source solutions can indeed compete with Big Tech’s black boxes.
In conclusion, OpenScholar is more than just a tool; it’s a beacon of hope for researchers navigating the turbulent waters of scientific literature. With its ability to synthesize vast amounts of information accurately and efficiently, it promises to transform the landscape of scientific research. As we embrace this new dawn, the future of research looks brighter than ever. OpenScholar is not just rewriting the rules; it’s setting a new standard for how we understand and engage with science.
Imagine a world where researchers can navigate through 45 million academic papers in mere seconds. OpenScholar’s retrieval-augmented language model does just that. It doesn’t simply regurgitate pre-trained knowledge like its proprietary counterparts, such as GPT-4o. Instead, it actively retrieves relevant studies, synthesizes their findings, and delivers answers grounded in real literature. This ability to stay anchored in verifiable sources sets OpenScholar apart.
In a recent benchmark test called ScholarQABench, OpenScholar outperformed larger models like GPT-4o in factuality and citation accuracy. While GPT-4o often fabricates citations—over 90% of the time in biomedical queries—OpenScholar remains steadfast, relying on legitimate sources. This reliability is crucial in a field where accuracy can mean the difference between life and death.
OpenScholar’s self-feedback inference loop iteratively refines its outputs, enhancing quality and incorporating new information. This dynamic process allows researchers to receive accurate, citation-backed answers to complex questions. The implications are profound. OpenScholar could accelerate scientific discovery, enabling experts to synthesize knowledge faster and with greater confidence.
But OpenScholar isn’t just about performance; it’s about accessibility. In a landscape dominated by expensive, closed systems, OpenScholar flips the script. It’s fully open-source, offering not just the code but the entire retrieval pipeline. This democratization of technology means smaller institutions and underfunded labs can access powerful AI tools without breaking the bank. The researchers estimate that OpenScholar is 100 times cheaper to operate than its proprietary counterparts.
However, OpenScholar is not without its limitations. Its reliance on open-access papers means it may miss critical findings locked behind paywalls. This gap is particularly concerning in high-stakes fields like medicine, where much research remains inaccessible. The developers acknowledge this shortcoming and hope future iterations can responsibly incorporate closed-access content.
Despite these challenges, OpenScholar represents a significant shift in scientific computing. It’s not just about engaging in conversation; it’s about processing, understanding, and synthesizing scientific literature with near-human accuracy. The numbers speak volumes. OpenScholar’s 8-billion-parameter model not only outperforms GPT-4o but also matches human experts in citation accuracy, where other AIs fail dramatically.
The emergence of OpenScholar raises important questions about the role of AI in science. While it excels in synthesizing literature, it’s not infallible. In expert evaluations, OpenScholar’s answers were preferred over human-written responses 70% of the time. The remaining 30% highlighted areas for improvement, such as failing to cite foundational papers. This underscores a vital truth: AI tools are meant to augment, not replace, human expertise.
Critics may argue that OpenScholar’s limitations hinder its utility in certain fields. However, its strengths lie in its ability to assist researchers in handling the time-consuming task of literature synthesis. This allows scientists to focus on interpretation and advancing knowledge rather than getting lost in the data deluge.
OpenScholar’s debut comes at a crucial time. The AI ecosystem is increasingly dominated by proprietary systems that are expensive and opaque. OpenScholar’s open-source model not only challenges this status quo but also provides a pathway for innovation. By releasing everything from code to training recipes, the developers are betting that openness will accelerate progress more than keeping breakthroughs behind closed doors.
As we stand on the brink of a new era in AI-assisted research, the bottleneck in scientific progress may no longer be our ability to process existing knowledge. Instead, it may hinge on our capacity to ask the right questions. OpenScholar is a testament to this shift, demonstrating that open-source solutions can indeed compete with Big Tech’s black boxes.
In conclusion, OpenScholar is more than just a tool; it’s a beacon of hope for researchers navigating the turbulent waters of scientific literature. With its ability to synthesize vast amounts of information accurately and efficiently, it promises to transform the landscape of scientific research. As we embrace this new dawn, the future of research looks brighter than ever. OpenScholar is not just rewriting the rules; it’s setting a new standard for how we understand and engage with science.