Navigating the Future of Network Operations Observability and RAG: A Dual Perspective
November 8, 2024, 7:28 pm
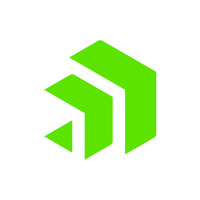
Location: United States, Massachusetts, Bedford
Employees: 1001-5000
Founded date: 2010
Total raised: $10.9M
In the fast-paced world of IT, clarity is gold. Two recent reports shine a light on critical trends: the EMA Radar Report on Network Operations Observability (NOO) and the complexities of Retrieval-Augmented Generation (RAG). Both concepts are vital for modern enterprises, yet they come with their own sets of challenges and opportunities.
The EMA Radar Report, released on November 6, 2024, evaluates 14 leading vendors in the NOO space. This report is a treasure map for IT organizations seeking to enhance their network management capabilities. It dives deep into the performance of various vendors, offering a comprehensive analysis that goes beyond mere data collection. It emphasizes actionable insights, a necessity in today’s data-driven landscape.
Network operations observability is not just a buzzword; it’s a lifeline for IT departments. As networks grow more complex, the need for robust monitoring tools becomes paramount. NOO solutions help organizations discover, monitor, troubleshoot, and plan capacity for their networks. They are the eyes and ears of IT infrastructure, providing a panoramic view of network health.
The report categorizes vendors based on key performance indicators (KPIs). It’s like a race, where each vendor’s capabilities are measured against a strict set of criteria. The vendors evaluated include industry giants like Cisco, IBM, and SolarWinds. Each has its strengths and weaknesses, but the report provides a clear picture of who leads the pack.
What sets this report apart is its focus on innovation. The market is evolving. Vendors are no longer just collecting data; they are harnessing advanced analytics, AI, and automation. This shift is crucial. IT organizations need tools that not only monitor but also predict and automate responses. The report reflects this evolution, showcasing how vendors are adapting to meet the demands of modern enterprises.
In contrast, the complexities of RAG present a different challenge. RAG is becoming the gold standard for enhancing the performance of large language models (LLMs). However, it’s not without its pitfalls. Increased data traffic, information overload, and data quality risks loom large. RAG promises improved accuracy and precision, but it requires careful implementation.
A recent webinar highlighted the nuances of RAG. Experts discussed strategies to maximize its potential while mitigating risks. The digital workplace is facing a knowledge crisis, and RAG could be the key to overcoming it. But, as with any tool, it’s only as good as its implementation.
The first step in successful RAG adoption is data enrichment. Context is king. Without it, AI models can falter. Controlled vocabularies, metadata enrichment, and knowledge graphs are essential for creating a rich context for GenAI applications. This is where the magic happens. When data is contextualized, the insights generated become more relevant and actionable.
Identifying data risks is another critical step. Enterprises must sift through their data to eliminate duplicates, inaccuracies, and compliance issues. It’s like cleaning a house before inviting guests. A cluttered data environment can lead to poor RAG outputs, which can hinder business success.
Quality control is paramount. Setting up filters to catch contradictory or biased content is essential. Monitoring RAG outputs for inaccuracies ensures that the insights generated are trustworthy. Regular reviews and user feedback mechanisms can help maintain quality. This is the backbone of a successful RAG strategy.
The choice of RAG implementation also matters. Will an organization use a vector database for retrieval or a RAG API? This decision can dictate the success of RAG within an enterprise. Tools like Coveo’s Relevance Generative Answering (RGA) offer robust solutions, combining RAG with AI relevancy to generate personalized and secure answers.
Contextual understanding is crucial in the world of RAG. As highlighted in the webinar, the meaning of information can change based on context. For instance, the word “gas” can refer to fuel, a chemical compound, or even a colloquial term. Understanding these nuances is vital for accurate retrieval and response generation.
Progress Semaphore emphasizes the importance of semantic context. By aligning implicit and explicit information, they create a rich metadata system. This allows LLMs to deliver answers that encapsulate the dynamic contexts within an enterprise. It’s about weaving a tapestry of information that reflects the organization’s unique landscape.
In conclusion, both Network Operations Observability and Retrieval-Augmented Generation are critical for modern enterprises. The EMA Radar Report provides a roadmap for navigating the NOO landscape, while the complexities of RAG highlight the need for careful implementation. As organizations strive for efficiency and accuracy, understanding these tools will be key to unlocking their full potential. The future is bright, but it requires a clear vision and strategic execution. Embrace the journey.
The EMA Radar Report, released on November 6, 2024, evaluates 14 leading vendors in the NOO space. This report is a treasure map for IT organizations seeking to enhance their network management capabilities. It dives deep into the performance of various vendors, offering a comprehensive analysis that goes beyond mere data collection. It emphasizes actionable insights, a necessity in today’s data-driven landscape.
Network operations observability is not just a buzzword; it’s a lifeline for IT departments. As networks grow more complex, the need for robust monitoring tools becomes paramount. NOO solutions help organizations discover, monitor, troubleshoot, and plan capacity for their networks. They are the eyes and ears of IT infrastructure, providing a panoramic view of network health.
The report categorizes vendors based on key performance indicators (KPIs). It’s like a race, where each vendor’s capabilities are measured against a strict set of criteria. The vendors evaluated include industry giants like Cisco, IBM, and SolarWinds. Each has its strengths and weaknesses, but the report provides a clear picture of who leads the pack.
What sets this report apart is its focus on innovation. The market is evolving. Vendors are no longer just collecting data; they are harnessing advanced analytics, AI, and automation. This shift is crucial. IT organizations need tools that not only monitor but also predict and automate responses. The report reflects this evolution, showcasing how vendors are adapting to meet the demands of modern enterprises.
In contrast, the complexities of RAG present a different challenge. RAG is becoming the gold standard for enhancing the performance of large language models (LLMs). However, it’s not without its pitfalls. Increased data traffic, information overload, and data quality risks loom large. RAG promises improved accuracy and precision, but it requires careful implementation.
A recent webinar highlighted the nuances of RAG. Experts discussed strategies to maximize its potential while mitigating risks. The digital workplace is facing a knowledge crisis, and RAG could be the key to overcoming it. But, as with any tool, it’s only as good as its implementation.
The first step in successful RAG adoption is data enrichment. Context is king. Without it, AI models can falter. Controlled vocabularies, metadata enrichment, and knowledge graphs are essential for creating a rich context for GenAI applications. This is where the magic happens. When data is contextualized, the insights generated become more relevant and actionable.
Identifying data risks is another critical step. Enterprises must sift through their data to eliminate duplicates, inaccuracies, and compliance issues. It’s like cleaning a house before inviting guests. A cluttered data environment can lead to poor RAG outputs, which can hinder business success.
Quality control is paramount. Setting up filters to catch contradictory or biased content is essential. Monitoring RAG outputs for inaccuracies ensures that the insights generated are trustworthy. Regular reviews and user feedback mechanisms can help maintain quality. This is the backbone of a successful RAG strategy.
The choice of RAG implementation also matters. Will an organization use a vector database for retrieval or a RAG API? This decision can dictate the success of RAG within an enterprise. Tools like Coveo’s Relevance Generative Answering (RGA) offer robust solutions, combining RAG with AI relevancy to generate personalized and secure answers.
Contextual understanding is crucial in the world of RAG. As highlighted in the webinar, the meaning of information can change based on context. For instance, the word “gas” can refer to fuel, a chemical compound, or even a colloquial term. Understanding these nuances is vital for accurate retrieval and response generation.
Progress Semaphore emphasizes the importance of semantic context. By aligning implicit and explicit information, they create a rich metadata system. This allows LLMs to deliver answers that encapsulate the dynamic contexts within an enterprise. It’s about weaving a tapestry of information that reflects the organization’s unique landscape.
In conclusion, both Network Operations Observability and Retrieval-Augmented Generation are critical for modern enterprises. The EMA Radar Report provides a roadmap for navigating the NOO landscape, while the complexities of RAG highlight the need for careful implementation. As organizations strive for efficiency and accuracy, understanding these tools will be key to unlocking their full potential. The future is bright, but it requires a clear vision and strategic execution. Embrace the journey.