The Rise of Intelligent AI: Navigating Complexity with Precision
November 5, 2024, 3:36 am
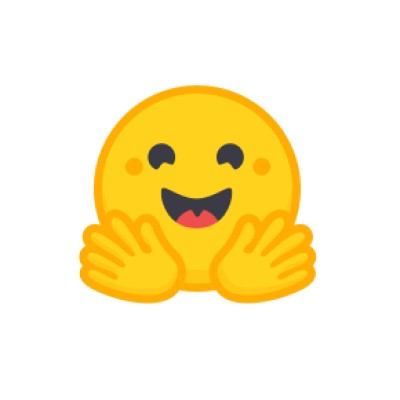
Location: Australia, New South Wales, Concord
Employees: 51-200
Founded date: 2016
Total raised: $494M
Artificial intelligence is evolving. It’s no longer just about brute force or sheer size. Two recent breakthroughs highlight this shift: Microsoft’s OmniParser and a collaborative effort from UC San Diego and Tsinghua University. Both innovations illustrate a growing understanding of how AI can operate more intelligently in complex environments.
First, let’s delve into OmniParser. Released by Microsoft, this open-source tool is designed to transform screenshots into structured data. Imagine a translator for visual information. It takes the chaos of a screen and organizes it into something an AI can understand. This is crucial as AI systems increasingly need to interact with graphical user interfaces (GUIs).
OmniParser is a game-changer. It uses advanced object detection and optical character recognition (OCR) to identify elements on a screen. Think of it as a digital eye, capable of spotting buttons, text, and icons. Once identified, these elements are converted into structured data that AI models, like GPT-4V, can act upon. This allows AI to perform tasks autonomously, from filling out forms to navigating complex applications.
The technology behind OmniParser is a symphony of various AI models. YOLOv8 detects interactable elements, while BLIP-2 provides context. Finally, GPT-4V makes decisions based on this information. This collaboration enables a seamless interaction with diverse GUIs, making OmniParser a versatile tool in the AI toolkit.
What sets OmniParser apart is its open-source nature. Developers can access and modify it, fostering a community-driven approach to improvement. This flexibility invites experimentation, allowing the model to evolve rapidly. The presence of OmniParser on platforms like Hugging Face has made it accessible to a wide audience, accelerating its adoption.
However, challenges remain. OmniParser struggles with repeated icons, often misidentifying their functions. The OCR component can also falter, particularly with overlapping text. These limitations highlight the complexities of designing AI that can navigate intricate screen environments. Yet, the optimism within the AI community suggests that these issues will be addressed through ongoing collaboration and refinement.
Now, let’s shift gears to the research from UC San Diego and Tsinghua University. Their work focuses on teaching AI when to rely on internal knowledge versus external tools. This method, dubbed “Adapting While Learning,” mimics human problem-solving. Just as a seasoned expert knows when to consult a manual, AI can learn to assess problem complexity and decide whether to seek help.
The results are impressive. The researchers achieved a 28% improvement in accuracy by training a smaller model—just 8 billion parameters—compared to larger counterparts. This challenges the prevailing notion that bigger is always better in AI. Instead, it emphasizes the importance of strategic decision-making.
The two-step training process involves “World Knowledge Distillation” and “Tool Usage Adaptation.” In the first phase, the model learns from solutions generated using external tools. In the second, it categorizes problems as “easy” or “hard,” deciding when to use tools accordingly. This approach not only enhances efficiency but also reduces computational costs, a significant concern for businesses deploying AI.
The implications of this research are profound. By teaching AI to make human-like decisions about tool usage, organizations can streamline operations. This is particularly valuable in fields like scientific research, where precision is paramount. The ability to discern when to seek help can prevent costly errors and improve overall outcomes.
Both OmniParser and the UC San Diego research signify a shift in AI development. They illustrate a move towards smarter, more efficient systems that prioritize understanding and adaptability. This is a departure from the traditional focus on size and power.
As AI continues to integrate into various sectors, the ability to navigate complexity with precision will be crucial. Whether it’s parsing a screen or deciding when to ask for help, these advancements pave the way for a more intelligent future.
In essence, the future of AI lies not just in its capabilities but in its wisdom. Knowing when to act and when to seek assistance is a hallmark of intelligence. As these technologies evolve, they will not only enhance efficiency but also foster a deeper understanding of the tasks at hand.
The journey of AI is just beginning. With tools like OmniParser and innovative research paving the way, we are on the brink of a new era. An era where AI is not just a tool but a thoughtful partner in navigating the complexities of our digital world. The horizon is bright, and the possibilities are endless.
First, let’s delve into OmniParser. Released by Microsoft, this open-source tool is designed to transform screenshots into structured data. Imagine a translator for visual information. It takes the chaos of a screen and organizes it into something an AI can understand. This is crucial as AI systems increasingly need to interact with graphical user interfaces (GUIs).
OmniParser is a game-changer. It uses advanced object detection and optical character recognition (OCR) to identify elements on a screen. Think of it as a digital eye, capable of spotting buttons, text, and icons. Once identified, these elements are converted into structured data that AI models, like GPT-4V, can act upon. This allows AI to perform tasks autonomously, from filling out forms to navigating complex applications.
The technology behind OmniParser is a symphony of various AI models. YOLOv8 detects interactable elements, while BLIP-2 provides context. Finally, GPT-4V makes decisions based on this information. This collaboration enables a seamless interaction with diverse GUIs, making OmniParser a versatile tool in the AI toolkit.
What sets OmniParser apart is its open-source nature. Developers can access and modify it, fostering a community-driven approach to improvement. This flexibility invites experimentation, allowing the model to evolve rapidly. The presence of OmniParser on platforms like Hugging Face has made it accessible to a wide audience, accelerating its adoption.
However, challenges remain. OmniParser struggles with repeated icons, often misidentifying their functions. The OCR component can also falter, particularly with overlapping text. These limitations highlight the complexities of designing AI that can navigate intricate screen environments. Yet, the optimism within the AI community suggests that these issues will be addressed through ongoing collaboration and refinement.
Now, let’s shift gears to the research from UC San Diego and Tsinghua University. Their work focuses on teaching AI when to rely on internal knowledge versus external tools. This method, dubbed “Adapting While Learning,” mimics human problem-solving. Just as a seasoned expert knows when to consult a manual, AI can learn to assess problem complexity and decide whether to seek help.
The results are impressive. The researchers achieved a 28% improvement in accuracy by training a smaller model—just 8 billion parameters—compared to larger counterparts. This challenges the prevailing notion that bigger is always better in AI. Instead, it emphasizes the importance of strategic decision-making.
The two-step training process involves “World Knowledge Distillation” and “Tool Usage Adaptation.” In the first phase, the model learns from solutions generated using external tools. In the second, it categorizes problems as “easy” or “hard,” deciding when to use tools accordingly. This approach not only enhances efficiency but also reduces computational costs, a significant concern for businesses deploying AI.
The implications of this research are profound. By teaching AI to make human-like decisions about tool usage, organizations can streamline operations. This is particularly valuable in fields like scientific research, where precision is paramount. The ability to discern when to seek help can prevent costly errors and improve overall outcomes.
Both OmniParser and the UC San Diego research signify a shift in AI development. They illustrate a move towards smarter, more efficient systems that prioritize understanding and adaptability. This is a departure from the traditional focus on size and power.
As AI continues to integrate into various sectors, the ability to navigate complexity with precision will be crucial. Whether it’s parsing a screen or deciding when to ask for help, these advancements pave the way for a more intelligent future.
In essence, the future of AI lies not just in its capabilities but in its wisdom. Knowing when to act and when to seek assistance is a hallmark of intelligence. As these technologies evolve, they will not only enhance efficiency but also foster a deeper understanding of the tasks at hand.
The journey of AI is just beginning. With tools like OmniParser and innovative research paving the way, we are on the brink of a new era. An era where AI is not just a tool but a thoughtful partner in navigating the complexities of our digital world. The horizon is bright, and the possibilities are endless.