The Rise of Mamba: A New Contender in Deep Learning
November 1, 2024, 5:50 am
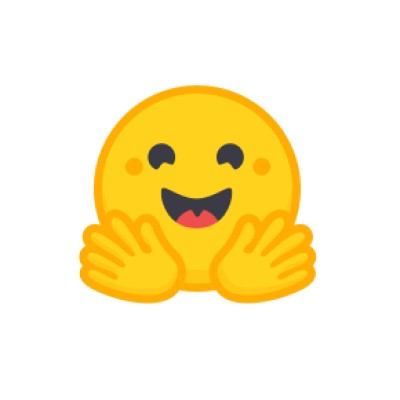
Location: Australia, New South Wales, Concord
Employees: 51-200
Founded date: 2016
Total raised: $494M
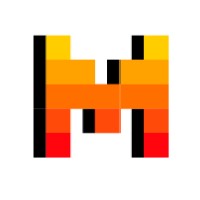
Location: France, Ile-de-France, Paris
Employees: 11-50
Founded date: 2023
Total raised: $1.26B
In the world of deep learning, transformers reign supreme. They’ve reshaped the landscape, powering everything from chatbots to complex language models. Yet, like a towering giant, they have their vulnerabilities. Enter Mamba, a new architecture designed to tackle the shortcomings of transformers.
Mamba emerged from the shadows in December 2023, promising to redefine how we approach language modeling. Its main advantage? A significant reduction in computational complexity. Traditional transformers suffer from quadratic scaling issues, meaning as input size grows, so do the demands on memory and processing power. Mamba flips this script, offering a more efficient alternative.
At its core, Mamba is built on the principles of State Space Models (SSM). These models allow for a more nuanced understanding of hidden states and predictions. Unlike recurrent neural networks (RNNs), which often forget earlier inputs, SSMs maintain a compact representation of the entire input history. This is akin to having a photographic memory rather than relying on fleeting snapshots.
The architecture of Mamba introduces several innovations. It combines three representations: continuous, recurrent, and convolutional. Each has its strengths and weaknesses. Continuous representations excel in capturing the dynamics of data over time, while recurrent models provide quick inference. Convolutional representations, on the other hand, leverage parallel processing capabilities of GPUs, enhancing training speed.
But Mamba doesn’t stop there. It incorporates selective scanning, allowing the model to filter out irrelevant information. This is crucial in tasks where not all input data holds significance. Imagine a librarian who can instantly identify which books are essential for a research project, ignoring the rest. This selective focus enhances the model's efficiency and accuracy.
Moreover, Mamba is designed with hardware efficiency in mind. It employs a unique algorithm that optimizes memory usage, making it suitable for deployment on various platforms. This is particularly important as the demand for accessible AI solutions grows. Mamba’s architecture can adapt to different hardware configurations, ensuring that it can run on everything from high-end servers to more modest setups.
The impact of Mamba is already being felt in the machine learning community. Its introduction has sparked a flurry of research and experimentation. Models like Jamba and FalconMamba are emerging, showcasing the versatility of Mamba’s architecture. The excitement is palpable, as researchers explore the potential of combining Mamba with existing transformer frameworks.
As we delve deeper into Mamba, it’s essential to understand its foundational elements. The architecture is built on the principles of linear state space layers. These layers allow for a dynamic approach to processing input data. By treating input sequences as discrete events, Mamba can efficiently manage and update its internal states. This is akin to a skilled chef who knows exactly when to add ingredients to a simmering pot, ensuring a perfect blend of flavors.
Mamba’s selective scanning mechanism is particularly noteworthy. It enables the model to adapt its parameters based on the input data. This means that the model can learn to prioritize certain pieces of information over others. For instance, in a sentence filled with filler words, Mamba can identify the key terms that carry the most weight. This capability is a game-changer for tasks like text summarization and information retrieval.
In practical applications, Mamba has shown promise in various domains. From natural language processing to image recognition, its versatility is becoming apparent. Researchers are beginning to test Mamba against established benchmarks, and early results indicate that it holds its own against traditional transformers.
However, the journey is just beginning. As with any new technology, challenges remain. The community is still exploring the best ways to integrate Mamba into existing workflows. There’s a learning curve involved, as practitioners adapt to the nuances of this new architecture. But the potential rewards are significant.
The future of Mamba looks bright. As more researchers adopt this architecture, we can expect to see a wave of innovations. The landscape of deep learning is evolving, and Mamba is at the forefront of this transformation. It represents a shift towards more efficient, adaptable, and powerful models.
In conclusion, Mamba is not just another contender in the deep learning arena; it’s a beacon of innovation. By addressing the limitations of transformers and introducing a more efficient architecture, Mamba is poised to change the game. As we continue to explore its capabilities, one thing is clear: the future of AI is here, and it’s called Mamba.
With its promise of efficiency and adaptability, Mamba could very well become the backbone of future AI applications. The excitement surrounding its development is a testament to the ever-evolving nature of technology. As we look ahead, the possibilities are endless. Mamba is not just a model; it’s a movement.
Mamba emerged from the shadows in December 2023, promising to redefine how we approach language modeling. Its main advantage? A significant reduction in computational complexity. Traditional transformers suffer from quadratic scaling issues, meaning as input size grows, so do the demands on memory and processing power. Mamba flips this script, offering a more efficient alternative.
At its core, Mamba is built on the principles of State Space Models (SSM). These models allow for a more nuanced understanding of hidden states and predictions. Unlike recurrent neural networks (RNNs), which often forget earlier inputs, SSMs maintain a compact representation of the entire input history. This is akin to having a photographic memory rather than relying on fleeting snapshots.
The architecture of Mamba introduces several innovations. It combines three representations: continuous, recurrent, and convolutional. Each has its strengths and weaknesses. Continuous representations excel in capturing the dynamics of data over time, while recurrent models provide quick inference. Convolutional representations, on the other hand, leverage parallel processing capabilities of GPUs, enhancing training speed.
But Mamba doesn’t stop there. It incorporates selective scanning, allowing the model to filter out irrelevant information. This is crucial in tasks where not all input data holds significance. Imagine a librarian who can instantly identify which books are essential for a research project, ignoring the rest. This selective focus enhances the model's efficiency and accuracy.
Moreover, Mamba is designed with hardware efficiency in mind. It employs a unique algorithm that optimizes memory usage, making it suitable for deployment on various platforms. This is particularly important as the demand for accessible AI solutions grows. Mamba’s architecture can adapt to different hardware configurations, ensuring that it can run on everything from high-end servers to more modest setups.
The impact of Mamba is already being felt in the machine learning community. Its introduction has sparked a flurry of research and experimentation. Models like Jamba and FalconMamba are emerging, showcasing the versatility of Mamba’s architecture. The excitement is palpable, as researchers explore the potential of combining Mamba with existing transformer frameworks.
As we delve deeper into Mamba, it’s essential to understand its foundational elements. The architecture is built on the principles of linear state space layers. These layers allow for a dynamic approach to processing input data. By treating input sequences as discrete events, Mamba can efficiently manage and update its internal states. This is akin to a skilled chef who knows exactly when to add ingredients to a simmering pot, ensuring a perfect blend of flavors.
Mamba’s selective scanning mechanism is particularly noteworthy. It enables the model to adapt its parameters based on the input data. This means that the model can learn to prioritize certain pieces of information over others. For instance, in a sentence filled with filler words, Mamba can identify the key terms that carry the most weight. This capability is a game-changer for tasks like text summarization and information retrieval.
In practical applications, Mamba has shown promise in various domains. From natural language processing to image recognition, its versatility is becoming apparent. Researchers are beginning to test Mamba against established benchmarks, and early results indicate that it holds its own against traditional transformers.
However, the journey is just beginning. As with any new technology, challenges remain. The community is still exploring the best ways to integrate Mamba into existing workflows. There’s a learning curve involved, as practitioners adapt to the nuances of this new architecture. But the potential rewards are significant.
The future of Mamba looks bright. As more researchers adopt this architecture, we can expect to see a wave of innovations. The landscape of deep learning is evolving, and Mamba is at the forefront of this transformation. It represents a shift towards more efficient, adaptable, and powerful models.
In conclusion, Mamba is not just another contender in the deep learning arena; it’s a beacon of innovation. By addressing the limitations of transformers and introducing a more efficient architecture, Mamba is poised to change the game. As we continue to explore its capabilities, one thing is clear: the future of AI is here, and it’s called Mamba.
With its promise of efficiency and adaptability, Mamba could very well become the backbone of future AI applications. The excitement surrounding its development is a testament to the ever-evolving nature of technology. As we look ahead, the possibilities are endless. Mamba is not just a model; it’s a movement.