Navigating the AI Cost Labyrinth: Strategies for Success in a Competitive Landscape
October 29, 2024, 7:04 pm
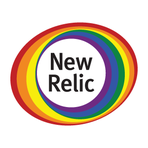
Location: Ireland, Dublin
Employees: 1001-5000
Founded date: 2008
Total raised: $329.5M
The AI revolution is upon us. It’s a whirlwind of innovation, competition, and unpredictability. Businesses across the globe are scrambling to harness the power of artificial intelligence. But with great power comes great expense. The cost of AI innovation is a complex puzzle, and companies must find ways to navigate this labyrinth to stay competitive.
The landscape is dominated by tech giants like OpenAI, Google, and Meta. They are racing to develop generative AI models that promise to transform industries. But for smaller enterprises, the stakes are high. The costs associated with AI tools can be staggering. Companies are not just investing in technology; they are investing in their future.
AI tools are not cheap. The price tags can be shocking. Companies developing AI features are discovering that cutting-edge services come with hefty costs. Models like OpenAI’s ChatGPT-4 Turbo and Google’s Gemini 1.5 Pro demand significant financial resources. Even newer models, which promise better performance at lower costs, can lead to unexpected expenses. As businesses push these models into complex use cases, costs can spiral out of control.
Understanding the true cost of AI is crucial. Companies face three primary cost structures: self-hosted/open source, pay-as-you-go (PAYGO), and provisioned throughput units (PTU). Self-hosting can be a double-edged sword. It allows companies to avoid vendor lock-in but often leads to high operational costs. Open-source models offer a more affordable alternative, yet they struggle to match the performance of proprietary solutions.
The PAYGO model is appealing for experimentation. It charges based on tokens processed, making it suitable for limited workloads. However, as traffic scales, performance can become inconsistent. Companies may find themselves switching between different endpoints to maximize efficiency. PTU offers guaranteed performance but comes with steep costs. Striking a balance between these models is essential for financial sustainability.
So, how can companies control these unpredictable costs? First, narrowing the scope of AI features can significantly reduce spending. Focusing on use cases that provide the best value-to-LLM-query ratio is key. This requires creative engineering and iterative optimization. Companies must identify low-hanging fruit opportunities to minimize expensive calls to LLMs.
Next, businesses should choose models based on their specific needs rather than the latest trends. While staying at the forefront of AI technology is tempting, many companies can achieve their goals with slightly older models. These models can still deliver impressive results at a fraction of the cost. As AI technologies evolve, older models can be optimized to provide significant value.
Cost optimization is vital when moving AI applications from prototype to production. Cutting-edge models may be necessary during the prototyping phase, but they can be too costly for long-term use. After validating a concept, companies should focus on optimizing costs through improved prompting and retrieval-augmented generation. This ensures that AI applications remain effective and economically viable.
Visibility is another critical factor. Companies must understand how often they query an LLM and the associated costs. By controlling these queries and adopting AI-supportive techniques, businesses can predict and lower their AI expenses. Observability helps maintain reliability and efficiency throughout the AI technology stack. It provides the data needed to make informed decisions that limit expenses and maximize return on investment.
As the tech giants continue to battle for AI market leadership, the landscape will remain unpredictable. Companies must be realistic about their goals and needs. Those willing to step back from the cutting edge can benefit from more affordable AI services while still delivering value to their customers. Identifying the right use cases and leveraging emerging AI-supportive techniques will be crucial.
In this competitive environment, innovation is essential. New Relic, for example, has been recognized as a leader in Digital Experience Monitoring (DEM) by Gartner. Their integrated solution combines real user monitoring with application performance management, providing enterprises with an end-to-end view of customer experience. This holistic approach helps identify performance bottlenecks and optimize user paths.
New Relic’s commitment to innovation is evident in their recent enhancements. Features like session replay and mobile log management provide superior insights without the hassle of manual recording. This level of innovation positions New Relic as a key player in the observability space.
Customer feedback reinforces New Relic’s value. Users report achieving 100% uptime and gaining deep insights across their tech stacks. This level of satisfaction is crucial in a market where businesses rely on observability to tackle issues before they impact operations.
In conclusion, the cost of AI innovation is a multifaceted challenge. Companies must navigate this labyrinth with strategic foresight. By narrowing their focus, choosing the right models, optimizing costs, and embracing visibility, businesses can thrive in this competitive landscape. The future of AI is bright, but only for those who can master the art of balancing cost and performance. As the AI revolution continues to unfold, adaptability will be the key to success.
The landscape is dominated by tech giants like OpenAI, Google, and Meta. They are racing to develop generative AI models that promise to transform industries. But for smaller enterprises, the stakes are high. The costs associated with AI tools can be staggering. Companies are not just investing in technology; they are investing in their future.
AI tools are not cheap. The price tags can be shocking. Companies developing AI features are discovering that cutting-edge services come with hefty costs. Models like OpenAI’s ChatGPT-4 Turbo and Google’s Gemini 1.5 Pro demand significant financial resources. Even newer models, which promise better performance at lower costs, can lead to unexpected expenses. As businesses push these models into complex use cases, costs can spiral out of control.
Understanding the true cost of AI is crucial. Companies face three primary cost structures: self-hosted/open source, pay-as-you-go (PAYGO), and provisioned throughput units (PTU). Self-hosting can be a double-edged sword. It allows companies to avoid vendor lock-in but often leads to high operational costs. Open-source models offer a more affordable alternative, yet they struggle to match the performance of proprietary solutions.
The PAYGO model is appealing for experimentation. It charges based on tokens processed, making it suitable for limited workloads. However, as traffic scales, performance can become inconsistent. Companies may find themselves switching between different endpoints to maximize efficiency. PTU offers guaranteed performance but comes with steep costs. Striking a balance between these models is essential for financial sustainability.
So, how can companies control these unpredictable costs? First, narrowing the scope of AI features can significantly reduce spending. Focusing on use cases that provide the best value-to-LLM-query ratio is key. This requires creative engineering and iterative optimization. Companies must identify low-hanging fruit opportunities to minimize expensive calls to LLMs.
Next, businesses should choose models based on their specific needs rather than the latest trends. While staying at the forefront of AI technology is tempting, many companies can achieve their goals with slightly older models. These models can still deliver impressive results at a fraction of the cost. As AI technologies evolve, older models can be optimized to provide significant value.
Cost optimization is vital when moving AI applications from prototype to production. Cutting-edge models may be necessary during the prototyping phase, but they can be too costly for long-term use. After validating a concept, companies should focus on optimizing costs through improved prompting and retrieval-augmented generation. This ensures that AI applications remain effective and economically viable.
Visibility is another critical factor. Companies must understand how often they query an LLM and the associated costs. By controlling these queries and adopting AI-supportive techniques, businesses can predict and lower their AI expenses. Observability helps maintain reliability and efficiency throughout the AI technology stack. It provides the data needed to make informed decisions that limit expenses and maximize return on investment.
As the tech giants continue to battle for AI market leadership, the landscape will remain unpredictable. Companies must be realistic about their goals and needs. Those willing to step back from the cutting edge can benefit from more affordable AI services while still delivering value to their customers. Identifying the right use cases and leveraging emerging AI-supportive techniques will be crucial.
In this competitive environment, innovation is essential. New Relic, for example, has been recognized as a leader in Digital Experience Monitoring (DEM) by Gartner. Their integrated solution combines real user monitoring with application performance management, providing enterprises with an end-to-end view of customer experience. This holistic approach helps identify performance bottlenecks and optimize user paths.
New Relic’s commitment to innovation is evident in their recent enhancements. Features like session replay and mobile log management provide superior insights without the hassle of manual recording. This level of innovation positions New Relic as a key player in the observability space.
Customer feedback reinforces New Relic’s value. Users report achieving 100% uptime and gaining deep insights across their tech stacks. This level of satisfaction is crucial in a market where businesses rely on observability to tackle issues before they impact operations.
In conclusion, the cost of AI innovation is a multifaceted challenge. Companies must navigate this labyrinth with strategic foresight. By narrowing their focus, choosing the right models, optimizing costs, and embracing visibility, businesses can thrive in this competitive landscape. The future of AI is bright, but only for those who can master the art of balancing cost and performance. As the AI revolution continues to unfold, adaptability will be the key to success.