The Evolution of Language Model Benchmarking: A New Era with MERA v.1.2.0
October 1, 2024, 9:38 am
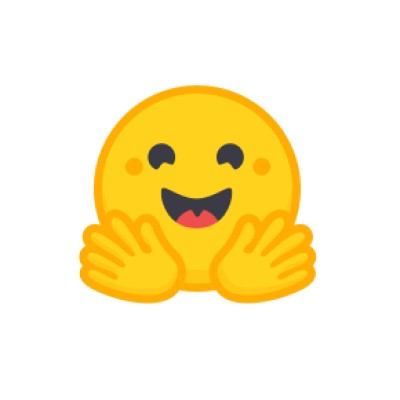
Location: Australia, New South Wales, Concord
Employees: 51-200
Founded date: 2016
Total raised: $494M
In the rapidly evolving landscape of artificial intelligence, language models (LLMs) are the stars of the show. They are the titans of technology, boasting billions of parameters and the ability to perform complex tasks. However, with great power comes great responsibility. As these models become more prevalent, the need for reliable benchmarking becomes critical. Enter MERA v.1.2.0, a significant update to an independent benchmarking platform that aims to set new standards in evaluating LLMs.
MERA, which stands for Model Evaluation and Ranking Assessment, first emerged last year as a response to the growing demand for a robust framework to assess the capabilities of large language models. The initial version laid the groundwork, but the latest update promises to refine and enhance the benchmarking process significantly.
The new version introduces a dynamic leaderboard featuring over 50 models, an updated codebase, and improved datasets. This is not just an incremental update; it’s a leap forward. The MERA team has listened to feedback from the NLP community, incorporating suggestions and criticisms to create a more effective evaluation tool.
At its core, MERA v.1.2.0 is designed to provide a comprehensive assessment of LLMs. The platform evaluates models across various tasks, ensuring that the results are not only accurate but also reflective of real-world applications. The new dynamic leaderboard allows users to filter results based on model characteristics, evaluation methods, and specific datasets. This flexibility is crucial for researchers and developers who need to understand how their models stack up against the competition.
One of the standout features of this update is the introduction of a new dataset, the Massive Multitask Russian AMplified Understudy (MaMuRAMu). This dataset is tailored for the Russian language, expanding MERA's reach and utility. It reflects the platform's commitment to inclusivity and diversity in language processing, recognizing that the world of AI is not limited to English.
The updated codebase also enhances the benchmarking process. By synchronizing with the latest version of the lm-evaluation-harness framework, MERA ensures that it remains at the forefront of technological advancements. This synchronization allows for the integration of new evaluation strategies and metrics, which are essential for keeping pace with the rapid development of LLMs.
MERA v.1.2.0 also addresses some of the limitations of its predecessor. The previous version struggled with certain models due to API constraints, but the new update allows for broader compatibility. This means that models like OpenAI's GPT-4 can now be evaluated, providing a more comprehensive picture of the landscape.
The importance of benchmarking cannot be overstated. As LLMs become more integrated into applications ranging from customer service to content creation, ensuring their reliability and accuracy is paramount. The risks associated with poorly performing models can lead to misinformation, user dissatisfaction, and even ethical concerns. Therefore, a robust benchmarking framework like MERA is essential for instilling confidence in these technologies.
Moreover, the update emphasizes the significance of prompt engineering. The way prompts are structured can drastically affect the performance of LLMs. MERA v.1.2.0 introduces a refined approach to prompt selection, ensuring that evaluations are not unduly influenced by the wording of prompts. This is a critical step in creating a fair and accurate assessment environment.
The platform also acknowledges the diverse nature of LLMs. By separating results for different types of models—such as those trained with supervised fine-tuning (SFT) versus pre-trained models—MERA allows for more nuanced comparisons. This differentiation is vital for understanding how various training methodologies impact model performance.
In addition to these technical advancements, MERA v.1.2.0 enhances user experience. The submission process for model evaluations has been streamlined, making it easier for researchers to contribute their findings. The new user interface is designed for clarity and efficiency, ensuring that users can navigate the platform with ease.
As we look to the future, the implications of MERA's advancements are profound. The platform not only sets a new standard for benchmarking LLMs but also fosters a culture of transparency and collaboration within the AI community. By providing a reliable framework for evaluation, MERA encourages developers to push the boundaries of what is possible with language models.
In conclusion, MERA v.1.2.0 represents a significant milestone in the journey of language model benchmarking. It combines technical innovation with a commitment to inclusivity and accuracy. As LLMs continue to evolve, platforms like MERA will play a crucial role in ensuring that these powerful tools are used responsibly and effectively. The future of AI is bright, and with robust benchmarking in place, we can navigate it with confidence.
MERA, which stands for Model Evaluation and Ranking Assessment, first emerged last year as a response to the growing demand for a robust framework to assess the capabilities of large language models. The initial version laid the groundwork, but the latest update promises to refine and enhance the benchmarking process significantly.
The new version introduces a dynamic leaderboard featuring over 50 models, an updated codebase, and improved datasets. This is not just an incremental update; it’s a leap forward. The MERA team has listened to feedback from the NLP community, incorporating suggestions and criticisms to create a more effective evaluation tool.
At its core, MERA v.1.2.0 is designed to provide a comprehensive assessment of LLMs. The platform evaluates models across various tasks, ensuring that the results are not only accurate but also reflective of real-world applications. The new dynamic leaderboard allows users to filter results based on model characteristics, evaluation methods, and specific datasets. This flexibility is crucial for researchers and developers who need to understand how their models stack up against the competition.
One of the standout features of this update is the introduction of a new dataset, the Massive Multitask Russian AMplified Understudy (MaMuRAMu). This dataset is tailored for the Russian language, expanding MERA's reach and utility. It reflects the platform's commitment to inclusivity and diversity in language processing, recognizing that the world of AI is not limited to English.
The updated codebase also enhances the benchmarking process. By synchronizing with the latest version of the lm-evaluation-harness framework, MERA ensures that it remains at the forefront of technological advancements. This synchronization allows for the integration of new evaluation strategies and metrics, which are essential for keeping pace with the rapid development of LLMs.
MERA v.1.2.0 also addresses some of the limitations of its predecessor. The previous version struggled with certain models due to API constraints, but the new update allows for broader compatibility. This means that models like OpenAI's GPT-4 can now be evaluated, providing a more comprehensive picture of the landscape.
The importance of benchmarking cannot be overstated. As LLMs become more integrated into applications ranging from customer service to content creation, ensuring their reliability and accuracy is paramount. The risks associated with poorly performing models can lead to misinformation, user dissatisfaction, and even ethical concerns. Therefore, a robust benchmarking framework like MERA is essential for instilling confidence in these technologies.
Moreover, the update emphasizes the significance of prompt engineering. The way prompts are structured can drastically affect the performance of LLMs. MERA v.1.2.0 introduces a refined approach to prompt selection, ensuring that evaluations are not unduly influenced by the wording of prompts. This is a critical step in creating a fair and accurate assessment environment.
The platform also acknowledges the diverse nature of LLMs. By separating results for different types of models—such as those trained with supervised fine-tuning (SFT) versus pre-trained models—MERA allows for more nuanced comparisons. This differentiation is vital for understanding how various training methodologies impact model performance.
In addition to these technical advancements, MERA v.1.2.0 enhances user experience. The submission process for model evaluations has been streamlined, making it easier for researchers to contribute their findings. The new user interface is designed for clarity and efficiency, ensuring that users can navigate the platform with ease.
As we look to the future, the implications of MERA's advancements are profound. The platform not only sets a new standard for benchmarking LLMs but also fosters a culture of transparency and collaboration within the AI community. By providing a reliable framework for evaluation, MERA encourages developers to push the boundaries of what is possible with language models.
In conclusion, MERA v.1.2.0 represents a significant milestone in the journey of language model benchmarking. It combines technical innovation with a commitment to inclusivity and accuracy. As LLMs continue to evolve, platforms like MERA will play a crucial role in ensuring that these powerful tools are used responsibly and effectively. The future of AI is bright, and with robust benchmarking in place, we can navigate it with confidence.