The Cost of Conversational AI: A Deep Dive into Implementation and Value
September 16, 2024, 9:36 am
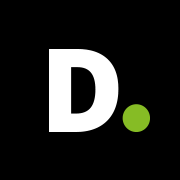
Location: Guatemala, Guatemala Department, Guatemala City
Employees: 10001+
Founded date: 1845
In the fast-paced world of technology, conversational AI stands out like a lighthouse in a storm. It promises to bridge the gap between businesses and customers, enhancing communication and driving efficiency. Yet, many organizations find themselves at a crossroads, unsure of how to implement this powerful tool. The decision to adopt conversational AI is not just about technology; it’s about understanding costs, benefits, and the long-term implications for the business.
Conversational AI has become a cornerstone for marketing, sales, and customer service. Over 40% of organizations in these sectors have embraced generative AI, making it the second most adopted technology after IT and cybersecurity. But as companies dive into this ocean of possibilities, they often face waves of uncertainty. Which large language model (LLM) should they choose? Should they go for open-source or closed-source solutions? And, most importantly, how much will it cost?
Many businesses opt for off-the-shelf conversational AI tools, but for those looking to integrate AI deeply into their operations, building an in-house solution may be the better route. This path, however, is fraught with challenges. The initial fear of costs can be paralyzing. To demystify this process, we’ll explore the costs associated with two leading LLMs: GPT-4o from OpenAI and Llama 3 from Meta.
**Understanding Costs: Setup and Processing**
When evaluating the costs of implementing conversational AI, two primary financial considerations emerge: setup costs and processing costs. Setup costs encompass everything needed to get the LLM operational, including development and operational expenses. Processing costs refer to the expenses incurred for each conversation once the AI is live.
The cost-to-value ratio varies significantly based on the intended use of the LLM. If speed is of the essence, a model like GPT-4o, which requires minimal setup, may be appealing. However, if a business anticipates high usage or desires greater control over its AI, investing in a more complex setup like Llama 3 could yield better long-term benefits.
**The Setup Dilemma**
GPT-4o is a closed-source model, meaning it’s hosted by OpenAI. This simplicity allows businesses to get started quickly with minimal setup. In contrast, Llama 3 is open-source, requiring companies to host the model on their own servers or through cloud providers. This approach offers more control but demands a greater initial investment in time and resources.
Cloud hosting costs can vary widely. Providers like AWS charge based on server time, while others may bundle costs differently. For example, Amazon Bedrock calculates fees based on the number of tokens processed, which can be advantageous for businesses with lower usage volumes.
**Processing Costs: The Heart of the Matter**
Once the LLM is operational, understanding the cost per conversation becomes crucial. Tokens serve as the currency in this realm. For instance, GPT-4o charges $0.005 per 1,000 input tokens and $0.015 per 1,000 output tokens. In a typical conversation, this can add up quickly.
Conversely, Llama 3 offers a more cost-effective solution, charging $0.00265 for input tokens and $0.00350 for output tokens. This means that, while GPT-4o may have lower upfront costs, Llama 3 can be nearly 50% cheaper per conversation once fully operational.
**Long-Term Considerations**
While initial costs are essential, businesses must also consider long-term implications. If a company plans to leverage conversational AI as a core service, the investment in building an in-house solution may pay off. However, for those who view AI as a supplementary tool, off-the-shelf products might suffice.
The choice between GPT-4o and Llama 3 also hinges on the level of control desired. Open-source models like Llama 3 provide flexibility and customization, which can be invaluable for businesses with specific needs. However, this comes at the cost of increased complexity and potential maintenance challenges.
**Navigating the Future**
As organizations weigh their options, it’s vital to remember that the landscape of conversational AI is continually evolving. New models and technologies emerge regularly, each promising to enhance efficiency and effectiveness. Staying informed and adaptable is key.
Moreover, the integration of conversational AI is not merely a technical challenge; it’s a strategic decision. Companies must align their AI initiatives with broader business goals. This alignment ensures that the technology serves as a catalyst for growth rather than a costly distraction.
**Conclusion: Making the Right Choice**
In the end, the decision to implement conversational AI is a journey, not a destination. It requires careful consideration of costs, benefits, and the unique needs of the business. Whether opting for a closed-source solution like GPT-4o or an open-source alternative like Llama 3, organizations must remain focused on their long-term objectives.
Conversational AI has the potential to transform customer interactions, streamline operations, and drive revenue. But like any powerful tool, it must be wielded wisely. By understanding the costs and implications of their choices, businesses can navigate the waters of AI with confidence, ensuring they emerge not just afloat, but thriving in the digital age.
Conversational AI has become a cornerstone for marketing, sales, and customer service. Over 40% of organizations in these sectors have embraced generative AI, making it the second most adopted technology after IT and cybersecurity. But as companies dive into this ocean of possibilities, they often face waves of uncertainty. Which large language model (LLM) should they choose? Should they go for open-source or closed-source solutions? And, most importantly, how much will it cost?
Many businesses opt for off-the-shelf conversational AI tools, but for those looking to integrate AI deeply into their operations, building an in-house solution may be the better route. This path, however, is fraught with challenges. The initial fear of costs can be paralyzing. To demystify this process, we’ll explore the costs associated with two leading LLMs: GPT-4o from OpenAI and Llama 3 from Meta.
**Understanding Costs: Setup and Processing**
When evaluating the costs of implementing conversational AI, two primary financial considerations emerge: setup costs and processing costs. Setup costs encompass everything needed to get the LLM operational, including development and operational expenses. Processing costs refer to the expenses incurred for each conversation once the AI is live.
The cost-to-value ratio varies significantly based on the intended use of the LLM. If speed is of the essence, a model like GPT-4o, which requires minimal setup, may be appealing. However, if a business anticipates high usage or desires greater control over its AI, investing in a more complex setup like Llama 3 could yield better long-term benefits.
**The Setup Dilemma**
GPT-4o is a closed-source model, meaning it’s hosted by OpenAI. This simplicity allows businesses to get started quickly with minimal setup. In contrast, Llama 3 is open-source, requiring companies to host the model on their own servers or through cloud providers. This approach offers more control but demands a greater initial investment in time and resources.
Cloud hosting costs can vary widely. Providers like AWS charge based on server time, while others may bundle costs differently. For example, Amazon Bedrock calculates fees based on the number of tokens processed, which can be advantageous for businesses with lower usage volumes.
**Processing Costs: The Heart of the Matter**
Once the LLM is operational, understanding the cost per conversation becomes crucial. Tokens serve as the currency in this realm. For instance, GPT-4o charges $0.005 per 1,000 input tokens and $0.015 per 1,000 output tokens. In a typical conversation, this can add up quickly.
Conversely, Llama 3 offers a more cost-effective solution, charging $0.00265 for input tokens and $0.00350 for output tokens. This means that, while GPT-4o may have lower upfront costs, Llama 3 can be nearly 50% cheaper per conversation once fully operational.
**Long-Term Considerations**
While initial costs are essential, businesses must also consider long-term implications. If a company plans to leverage conversational AI as a core service, the investment in building an in-house solution may pay off. However, for those who view AI as a supplementary tool, off-the-shelf products might suffice.
The choice between GPT-4o and Llama 3 also hinges on the level of control desired. Open-source models like Llama 3 provide flexibility and customization, which can be invaluable for businesses with specific needs. However, this comes at the cost of increased complexity and potential maintenance challenges.
**Navigating the Future**
As organizations weigh their options, it’s vital to remember that the landscape of conversational AI is continually evolving. New models and technologies emerge regularly, each promising to enhance efficiency and effectiveness. Staying informed and adaptable is key.
Moreover, the integration of conversational AI is not merely a technical challenge; it’s a strategic decision. Companies must align their AI initiatives with broader business goals. This alignment ensures that the technology serves as a catalyst for growth rather than a costly distraction.
**Conclusion: Making the Right Choice**
In the end, the decision to implement conversational AI is a journey, not a destination. It requires careful consideration of costs, benefits, and the unique needs of the business. Whether opting for a closed-source solution like GPT-4o or an open-source alternative like Llama 3, organizations must remain focused on their long-term objectives.
Conversational AI has the potential to transform customer interactions, streamline operations, and drive revenue. But like any powerful tool, it must be wielded wisely. By understanding the costs and implications of their choices, businesses can navigate the waters of AI with confidence, ensuring they emerge not just afloat, but thriving in the digital age.