The Future of AI: Navigating the Landscape of Few-Shot Learning and Interpretability
September 11, 2024, 11:37 pm
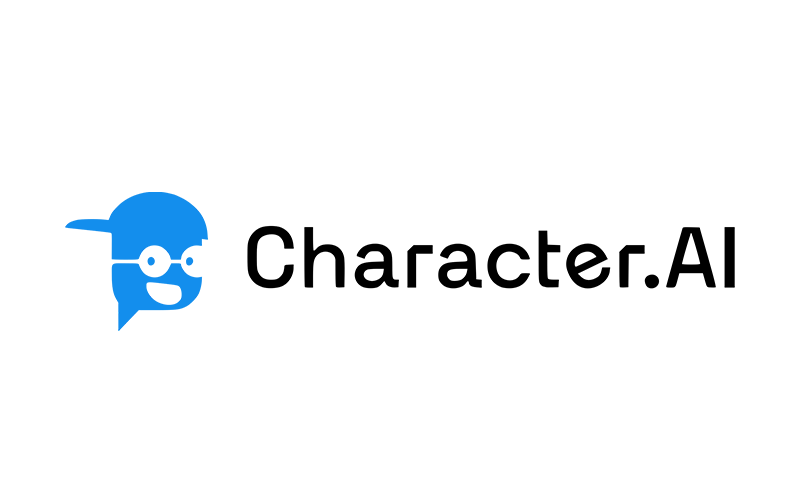
Location: Anguilla,
Employees: 11-50
Total raised: $150.5M
Artificial intelligence is evolving at breakneck speed. As we stand on the brink of a new era, two concepts emerge as pivotal: few-shot learning and interpretability. These ideas are not just buzzwords; they are the compass guiding us through the complex terrain of AI development.
Few-shot learning is like teaching a child to recognize animals with just a few pictures. Instead of bombarding the child with countless images, you show them a handful of examples. This method allows the child to generalize and identify new animals based on limited exposure. In the realm of AI, few-shot learning empowers large language models (LLMs) to adapt quickly to new tasks without extensive retraining. This efficiency is a game-changer.
Enter Prompt Poet, a tool that simplifies this process. Developed by Character.ai and now under Google's wing, Prompt Poet transforms the intricate art of prompt engineering into a user-friendly experience. It allows developers to create dynamic prompts that incorporate real-world data seamlessly. Imagine crafting a customer service chatbot that not only understands user queries but also responds in a brand's unique voice. This is the promise of Prompt Poet.
But why does this matter? As AI systems become more integrated into our daily lives, the ability to customize interactions is crucial. Few-shot learning enables businesses to tailor AI responses to their specific needs without the heavy lifting of model fine-tuning. This means faster deployment and more relevant interactions. The potential applications are vast, from customer service to education, where personalized learning experiences can be crafted with ease.
Yet, as we embrace these advancements, we must also confront the shadows lurking in the corners of AI development: interpretability. The question of how AI systems "think" is a complex puzzle. Are these models truly understanding the tasks they perform, or are they merely sophisticated parrots, regurgitating learned patterns without comprehension? This debate is akin to pondering whether a well-trained dog understands commands or simply responds to cues.
The challenge lies in the fact that AI models operate as black boxes. We can observe their outputs, but the inner workings remain obscured. Researchers are striving to peel back the layers of these black boxes, seeking to understand the algorithms that drive decision-making. This pursuit of interpretability is not just academic; it has real-world implications. As AI systems are deployed in critical areas like healthcare and finance, understanding their decision-making processes becomes paramount.
Consider the implications of a model that generates misleading information. If we cannot trace the source of its knowledge, how can we trust its outputs? This concern is amplified as AI systems become more autonomous. The fear is not unfounded; there have been instances where AI-generated content has led to misinformation. The need for transparency in AI is more pressing than ever.
Researchers are exploring mechanistic interpretability, a field that aims to uncover the logic behind AI decisions. By analyzing the weights and parameters of models, scientists hope to extract algorithms that can be understood and validated. This is akin to a mechanic diagnosing a car's issues by examining its parts. If we can identify how a model arrives at a conclusion, we can ensure its reliability.
However, the road to interpretability is fraught with challenges. The complexity of neural networks makes it difficult to pinpoint the exact reasons behind specific outputs. This is where the analogy of the brain comes into play. Just as neuroscientists study the brain's functions and disorders, AI researchers are delving into the intricacies of machine learning models. The goal is to bridge the gap between human understanding and machine logic.
As we navigate this landscape, the stakes are high. The integration of AI into society raises ethical questions. If AI systems can influence opinions and decisions, how do we ensure they align with human values? The potential for misuse is a constant concern. As AI becomes more prevalent in education, for instance, the risk of shaping young minds with biased information looms large.
The future of AI hinges on our ability to balance innovation with responsibility. Few-shot learning offers a pathway to create more adaptable and efficient systems, but without interpretability, we risk losing control over these powerful tools. The challenge is to develop AI that not only performs tasks effectively but also operates transparently.
In conclusion, the interplay between few-shot learning and interpretability will shape the future of AI. As we harness the power of these technologies, we must remain vigilant. The journey ahead is filled with promise, but it requires a commitment to understanding and accountability. The road to a responsible AI future is paved with knowledge, transparency, and ethical considerations. Let us tread carefully, for the implications of our choices will resonate for generations to come.
Few-shot learning is like teaching a child to recognize animals with just a few pictures. Instead of bombarding the child with countless images, you show them a handful of examples. This method allows the child to generalize and identify new animals based on limited exposure. In the realm of AI, few-shot learning empowers large language models (LLMs) to adapt quickly to new tasks without extensive retraining. This efficiency is a game-changer.
Enter Prompt Poet, a tool that simplifies this process. Developed by Character.ai and now under Google's wing, Prompt Poet transforms the intricate art of prompt engineering into a user-friendly experience. It allows developers to create dynamic prompts that incorporate real-world data seamlessly. Imagine crafting a customer service chatbot that not only understands user queries but also responds in a brand's unique voice. This is the promise of Prompt Poet.
But why does this matter? As AI systems become more integrated into our daily lives, the ability to customize interactions is crucial. Few-shot learning enables businesses to tailor AI responses to their specific needs without the heavy lifting of model fine-tuning. This means faster deployment and more relevant interactions. The potential applications are vast, from customer service to education, where personalized learning experiences can be crafted with ease.
Yet, as we embrace these advancements, we must also confront the shadows lurking in the corners of AI development: interpretability. The question of how AI systems "think" is a complex puzzle. Are these models truly understanding the tasks they perform, or are they merely sophisticated parrots, regurgitating learned patterns without comprehension? This debate is akin to pondering whether a well-trained dog understands commands or simply responds to cues.
The challenge lies in the fact that AI models operate as black boxes. We can observe their outputs, but the inner workings remain obscured. Researchers are striving to peel back the layers of these black boxes, seeking to understand the algorithms that drive decision-making. This pursuit of interpretability is not just academic; it has real-world implications. As AI systems are deployed in critical areas like healthcare and finance, understanding their decision-making processes becomes paramount.
Consider the implications of a model that generates misleading information. If we cannot trace the source of its knowledge, how can we trust its outputs? This concern is amplified as AI systems become more autonomous. The fear is not unfounded; there have been instances where AI-generated content has led to misinformation. The need for transparency in AI is more pressing than ever.
Researchers are exploring mechanistic interpretability, a field that aims to uncover the logic behind AI decisions. By analyzing the weights and parameters of models, scientists hope to extract algorithms that can be understood and validated. This is akin to a mechanic diagnosing a car's issues by examining its parts. If we can identify how a model arrives at a conclusion, we can ensure its reliability.
However, the road to interpretability is fraught with challenges. The complexity of neural networks makes it difficult to pinpoint the exact reasons behind specific outputs. This is where the analogy of the brain comes into play. Just as neuroscientists study the brain's functions and disorders, AI researchers are delving into the intricacies of machine learning models. The goal is to bridge the gap between human understanding and machine logic.
As we navigate this landscape, the stakes are high. The integration of AI into society raises ethical questions. If AI systems can influence opinions and decisions, how do we ensure they align with human values? The potential for misuse is a constant concern. As AI becomes more prevalent in education, for instance, the risk of shaping young minds with biased information looms large.
The future of AI hinges on our ability to balance innovation with responsibility. Few-shot learning offers a pathway to create more adaptable and efficient systems, but without interpretability, we risk losing control over these powerful tools. The challenge is to develop AI that not only performs tasks effectively but also operates transparently.
In conclusion, the interplay between few-shot learning and interpretability will shape the future of AI. As we harness the power of these technologies, we must remain vigilant. The journey ahead is filled with promise, but it requires a commitment to understanding and accountability. The road to a responsible AI future is paved with knowledge, transparency, and ethical considerations. Let us tread carefully, for the implications of our choices will resonate for generations to come.