The Rise of Sparse Autoencoders: Bridging the Gap Between Performance and Interpretability in AI
September 1, 2024, 4:34 am
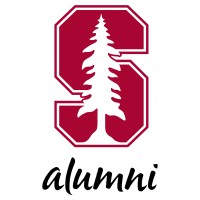
Location: United Kingdom, England, City of London
Employees: 10001+
Founded date: 1885
In the world of artificial intelligence, clarity often gets lost in complexity. The advent of deep learning, particularly with breakthroughs like AlexNet in 2012, marked a turning point. Models became powerful but opaque. They transformed into black boxes, making it difficult to decipher their decision-making processes. This lack of transparency poses significant challenges, especially in critical fields like healthcare and finance. Enter sparse autoencoders (SAEs), a promising solution to this growing problem.
Sparse autoencoders are a type of neural network architecture designed to enhance interpretability. Unlike traditional autoencoders, which compress input data into dense representations, SAEs focus on creating sparse representations. In these models, only a small fraction of neurons are active at any given time. This sparsity compels the network to learn clearer, more interpretable features. Each neuron captures specific, non-redundant information, making the model easier to understand.
The importance of sparse autoencoders extends beyond mere interpretability. Their inherent sparsity can reduce computational complexity and improve generalization. This efficiency makes them reliable across various applications. For instance, researchers have utilized SAEs to optimize feature extraction from brain data, aiding in the detection of autism spectrum disorders. This application exemplifies how SAEs can illuminate the inner workings of complex systems.
The approach taken by Anthropic, a leader in AI research, is particularly noteworthy. Their sparse autoencoders draw inspiration from biological neural networks, where only a subset of neurons is active at any moment. This design philosophy enhances efficiency and interpretability. Anthropic introduces innovative mechanisms to maximize the benefits of this sparsity.
One key technique employed in Anthropic's autoencoders is L1 regularization. This method adds a penalty to the loss function based on the absolute values of the weights. By doing so, the network is incentivized to minimize the number of active neurons. This balance between learning meaningful features and maintaining interpretability is crucial. The mathematical representation of this process highlights the elegance of the approach.
Another unique aspect of Anthropic's autoencoders is the use of structured sparsity. Unlike unstructured sparsity, where individual neurons are either active or inactive, structured sparsity groups neurons together. This grouping allows the network to learn more interpretable representations. For example, in a computer vision model, one group of neurons might specialize in edge detection, while another focuses on texture recognition. This organization not only enhances interpretability but also boosts the network's efficiency.
The application of sparse autoencoders follows a systematic process. The output of the model's activation layer is normalized and fed into the sparse autoencoder. This setup decomposes the activations into a linear combination of feature directions. The result is a model that not only interprets features but also predicts outcomes in a predictable manner. For instance, researchers can select a feature related to the Golden Gate Bridge and observe how the model generates text associated with it. This capability underscores the interpretability of the model's features.
Beyond interpretability, sparse autoencoders contribute to the reliability and ethical application of AI. By focusing on significant features and minimizing reliance on noisy or irrelevant data, these models are less likely to produce biased or unfair results. In credit scoring, for example, sparse autoencoders can ensure that decisions are based on relevant factors rather than spurious correlations. This aspect is vital for developing fair and unbiased AI systems, especially in sensitive areas where discrimination can have severe societal consequences.
In summary, the emergence of sparse autoencoders represents a significant advancement in AI. These models tackle the pressing issue of interpretability, making the inner workings of neural networks more comprehensible. From enhancing decision-making efficiency in critical sectors to promoting ethical AI deployment, sparse autoencoders demonstrate that performance and interpretability can coexist. They pave the way for powerful systems that are also transparent and trustworthy.
As AI continues to evolve, the importance of interpretability will only grow. Sparse autoencoders offer a glimpse into a future where complex models can be understood and trusted. This balance between power and clarity is essential for the responsible development of AI technologies. In a landscape where decisions can have profound impacts, the ability to interpret and understand AI systems is not just beneficial; it is imperative.
For those interested in the intersection of mathematics and AI, particularly in gaming and art, there are more insights to explore. The journey of understanding AI is just beginning, and sparse autoencoders are leading the way.
Sparse autoencoders are a type of neural network architecture designed to enhance interpretability. Unlike traditional autoencoders, which compress input data into dense representations, SAEs focus on creating sparse representations. In these models, only a small fraction of neurons are active at any given time. This sparsity compels the network to learn clearer, more interpretable features. Each neuron captures specific, non-redundant information, making the model easier to understand.
The importance of sparse autoencoders extends beyond mere interpretability. Their inherent sparsity can reduce computational complexity and improve generalization. This efficiency makes them reliable across various applications. For instance, researchers have utilized SAEs to optimize feature extraction from brain data, aiding in the detection of autism spectrum disorders. This application exemplifies how SAEs can illuminate the inner workings of complex systems.
The approach taken by Anthropic, a leader in AI research, is particularly noteworthy. Their sparse autoencoders draw inspiration from biological neural networks, where only a subset of neurons is active at any moment. This design philosophy enhances efficiency and interpretability. Anthropic introduces innovative mechanisms to maximize the benefits of this sparsity.
One key technique employed in Anthropic's autoencoders is L1 regularization. This method adds a penalty to the loss function based on the absolute values of the weights. By doing so, the network is incentivized to minimize the number of active neurons. This balance between learning meaningful features and maintaining interpretability is crucial. The mathematical representation of this process highlights the elegance of the approach.
Another unique aspect of Anthropic's autoencoders is the use of structured sparsity. Unlike unstructured sparsity, where individual neurons are either active or inactive, structured sparsity groups neurons together. This grouping allows the network to learn more interpretable representations. For example, in a computer vision model, one group of neurons might specialize in edge detection, while another focuses on texture recognition. This organization not only enhances interpretability but also boosts the network's efficiency.
The application of sparse autoencoders follows a systematic process. The output of the model's activation layer is normalized and fed into the sparse autoencoder. This setup decomposes the activations into a linear combination of feature directions. The result is a model that not only interprets features but also predicts outcomes in a predictable manner. For instance, researchers can select a feature related to the Golden Gate Bridge and observe how the model generates text associated with it. This capability underscores the interpretability of the model's features.
Beyond interpretability, sparse autoencoders contribute to the reliability and ethical application of AI. By focusing on significant features and minimizing reliance on noisy or irrelevant data, these models are less likely to produce biased or unfair results. In credit scoring, for example, sparse autoencoders can ensure that decisions are based on relevant factors rather than spurious correlations. This aspect is vital for developing fair and unbiased AI systems, especially in sensitive areas where discrimination can have severe societal consequences.
In summary, the emergence of sparse autoencoders represents a significant advancement in AI. These models tackle the pressing issue of interpretability, making the inner workings of neural networks more comprehensible. From enhancing decision-making efficiency in critical sectors to promoting ethical AI deployment, sparse autoencoders demonstrate that performance and interpretability can coexist. They pave the way for powerful systems that are also transparent and trustworthy.
As AI continues to evolve, the importance of interpretability will only grow. Sparse autoencoders offer a glimpse into a future where complex models can be understood and trusted. This balance between power and clarity is essential for the responsible development of AI technologies. In a landscape where decisions can have profound impacts, the ability to interpret and understand AI systems is not just beneficial; it is imperative.
For those interested in the intersection of mathematics and AI, particularly in gaming and art, there are more insights to explore. The journey of understanding AI is just beginning, and sparse autoencoders are leading the way.