The Dawn of 3D Generation: Nvidia's XCube Revolutionizes Digital Landscapes
August 29, 2024, 4:49 pm
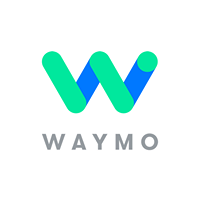
Location: United States, California, Mountain View
Employees: 1001-5000
Founded date: 2009
Total raised: $13.57B
In the realm of technology, innovation is the lifeblood. It fuels progress and shapes the future. Nvidia’s latest creation, XCube, is a testament to this relentless drive. This groundbreaking model for generating 3D objects is not just a step forward; it’s a leap into uncharted territory.
Imagine a world where entire cities can be conjured from thin air. Where intricate 3D models spring to life in seconds. This is the promise of XCube. It harnesses the power of Sparse Voxel Hierarchies, a method that transforms the way we think about 3D generation. Traditional models often stumble when faced with the challenges of resolution, efficiency, and scalability. XCube, however, dances gracefully through these hurdles.
At its core, XCube is a master of detail. It scales effortlessly from single objects to vast landscapes, achieving voxel resolutions that leave previous models in the dust. This isn’t just about creating pretty pictures; it’s about enhancing the very fabric of digital environments. The model supports user editing and universal attribute generation, making it a versatile tool for creators.
The journey to 3D generation begins with representation. Various methods have been explored: point clouds, triangular meshes, and neural implicit fields. Each has its strengths and weaknesses. Point clouds offer flexibility but falter in representing solid surfaces. Triangular meshes are bound by fixed topologies, while implicit fields struggle with the inductive bias needed for effective modeling. XCube aims to blend the best of these worlds, yet it faces its own challenges in resolution and scalability.
The methodology behind XCube is where the magic happens. It employs a hierarchical voxel diffusion process. This technique gradually refines coarse voxel grids into detailed structures. It’s a symphony of interconnected steps, starting with encoding 3D data into a sparse voxel hierarchy. The result? High-resolution 3D models enriched with attributes like surface normals and semantic labels.
XCube’s architecture is a marvel. It begins with a variational autoencoder (VAE) that compresses 3D data into a compact latent representation. This representation captures the essence of each voxel grid. The VAE operates independently at each voxel hierarchy level, ensuring that even the coarsest grids retain enough detail for refinement. This efficient encoding not only saves memory but accelerates training and inference.
The hierarchical nature of XCube allows it to manage varying levels of detail. It focuses computational resources on areas of interest, while less critical regions receive a lighter touch. This is akin to a painter who knows where to apply bold strokes and where to let the canvas breathe. The diffusion process, guided by stochastic noise reduction, transforms a noisy latent space into a cohesive 3D structure over several iterations.
Nvidia has put XCube to the test. They conducted experiments across various benchmarks, focusing on object-level and scene-level generation tasks. The results were impressive. XCube generated high-resolution 3D objects that outperformed previous models, particularly in categories like airplanes, cars, and chairs. It maintained high resolutions—up to 1024³ voxels—without sacrificing computational efficiency. The metrics spoke volumes: lower values in the 1-NNA, Chamfer Distance (CD), and Earth Mover's Distance (EMD) indicated a closer alignment with reality.
Human evaluations further validated XCube’s superiority. Participants overwhelmingly preferred the generated objects over those produced by competing methods. This human touch adds a layer of credibility to the model’s capabilities.
When it comes to scene generation, XCube excelled in complex environments. Tested on the Waymo Open Dataset and Karton City, it produced realistic urban landscapes filled with roads, buildings, and vehicles. The hierarchical approach allowed it to maintain fine details in critical areas while managing larger scenes effectively. Moreover, XCube demonstrated its prowess in completing scenes based on partial LiDAR data, showcasing its adaptability.
Looking ahead, XCube opens new avenues for research and development in generative 3D. The potential to integrate prompts from text or images could enhance its capabilities even further. The hierarchical approach could be expanded to tackle larger scales or more complex tasks, such as real-time 3D content generation. As the volume of 3D datasets grows, so too will the potential of models like XCube.
In a world where digital landscapes are becoming increasingly important, Nvidia’s XCube stands at the forefront. It’s not just a tool; it’s a gateway to new possibilities. The future of 3D generation is bright, and XCube is leading the charge. As we continue to explore the depths of digital creation, one thing is clear: the journey has only just begun. The horizon is vast, and the opportunities are limitless.
Imagine a world where entire cities can be conjured from thin air. Where intricate 3D models spring to life in seconds. This is the promise of XCube. It harnesses the power of Sparse Voxel Hierarchies, a method that transforms the way we think about 3D generation. Traditional models often stumble when faced with the challenges of resolution, efficiency, and scalability. XCube, however, dances gracefully through these hurdles.
At its core, XCube is a master of detail. It scales effortlessly from single objects to vast landscapes, achieving voxel resolutions that leave previous models in the dust. This isn’t just about creating pretty pictures; it’s about enhancing the very fabric of digital environments. The model supports user editing and universal attribute generation, making it a versatile tool for creators.
The journey to 3D generation begins with representation. Various methods have been explored: point clouds, triangular meshes, and neural implicit fields. Each has its strengths and weaknesses. Point clouds offer flexibility but falter in representing solid surfaces. Triangular meshes are bound by fixed topologies, while implicit fields struggle with the inductive bias needed for effective modeling. XCube aims to blend the best of these worlds, yet it faces its own challenges in resolution and scalability.
The methodology behind XCube is where the magic happens. It employs a hierarchical voxel diffusion process. This technique gradually refines coarse voxel grids into detailed structures. It’s a symphony of interconnected steps, starting with encoding 3D data into a sparse voxel hierarchy. The result? High-resolution 3D models enriched with attributes like surface normals and semantic labels.
XCube’s architecture is a marvel. It begins with a variational autoencoder (VAE) that compresses 3D data into a compact latent representation. This representation captures the essence of each voxel grid. The VAE operates independently at each voxel hierarchy level, ensuring that even the coarsest grids retain enough detail for refinement. This efficient encoding not only saves memory but accelerates training and inference.
The hierarchical nature of XCube allows it to manage varying levels of detail. It focuses computational resources on areas of interest, while less critical regions receive a lighter touch. This is akin to a painter who knows where to apply bold strokes and where to let the canvas breathe. The diffusion process, guided by stochastic noise reduction, transforms a noisy latent space into a cohesive 3D structure over several iterations.
Nvidia has put XCube to the test. They conducted experiments across various benchmarks, focusing on object-level and scene-level generation tasks. The results were impressive. XCube generated high-resolution 3D objects that outperformed previous models, particularly in categories like airplanes, cars, and chairs. It maintained high resolutions—up to 1024³ voxels—without sacrificing computational efficiency. The metrics spoke volumes: lower values in the 1-NNA, Chamfer Distance (CD), and Earth Mover's Distance (EMD) indicated a closer alignment with reality.
Human evaluations further validated XCube’s superiority. Participants overwhelmingly preferred the generated objects over those produced by competing methods. This human touch adds a layer of credibility to the model’s capabilities.
When it comes to scene generation, XCube excelled in complex environments. Tested on the Waymo Open Dataset and Karton City, it produced realistic urban landscapes filled with roads, buildings, and vehicles. The hierarchical approach allowed it to maintain fine details in critical areas while managing larger scenes effectively. Moreover, XCube demonstrated its prowess in completing scenes based on partial LiDAR data, showcasing its adaptability.
Looking ahead, XCube opens new avenues for research and development in generative 3D. The potential to integrate prompts from text or images could enhance its capabilities even further. The hierarchical approach could be expanded to tackle larger scales or more complex tasks, such as real-time 3D content generation. As the volume of 3D datasets grows, so too will the potential of models like XCube.
In a world where digital landscapes are becoming increasingly important, Nvidia’s XCube stands at the forefront. It’s not just a tool; it’s a gateway to new possibilities. The future of 3D generation is bright, and XCube is leading the charge. As we continue to explore the depths of digital creation, one thing is clear: the journey has only just begun. The horizon is vast, and the opportunities are limitless.