The Rise and Fall of AI: Unpacking the Strengths and Weaknesses of Language Models
August 13, 2024, 4:04 am
Google
Location: United States, New York
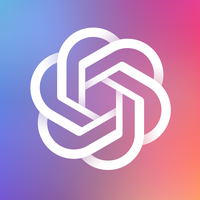
Location: United States, California, San Francisco
Employees: 201-500
Founded date: 2015
Total raised: $11.57B
In the fast-paced world of artificial intelligence, language models (LLMs) are the shining stars. They promise to revolutionize how we interact with technology. Yet, recent findings reveal cracks in their armor. Two recent developments highlight this duality: Alibaba Cloud's Qwen2-Math and a critical study exposing the limitations of LLMs in logical reasoning.
Alibaba Cloud has unveiled Qwen2-Math, a series of open-source LLMs designed to tackle mathematical problems. This initiative aims to bridge the gap between complex computations and user accessibility. The flagship model, Qwen2-Math-72B-Instruct, boasts an impressive accuracy rate of 84% on the MATH benchmark, which features 12,500 challenging math problems. This performance outshines competitors like OpenAI and Google, marking a significant leap in AI capabilities.
But the story doesn't end there. Qwen2-Math isn't just about numbers. It opens doors for startups, businesses, and academic institutions. The flexible licensing options make it an attractive tool for various applications. Even the smaller model, Qwen2-Math-1.5B, delivers results that rival larger counterparts. This suggests that size isn't everything; efficiency and training matter too.
The implications are vast. With plans to support multiple languages and enhance problem-solving algorithms, Qwen2-Math could democratize access to advanced mathematical tools. Imagine students in remote areas solving complex equations with the help of AI. The potential for educational reform is immense.
However, the excitement surrounding Qwen2-Math is tempered by a sobering reality. A recent study has exposed significant flaws in the logical reasoning capabilities of LLMs. Researchers found that even the most advanced models struggle with basic logical tasks. The study, using a simple problem involving Alice and her siblings, revealed that LLMs often fail to provide correct answers. In fact, more than half of the responses were incorrect.
This raises critical questions about the reliability of LLMs. If these models falter at elementary tasks, what does that mean for their application in more complex scenarios? The researchers noted that LLMs displayed overconfidence in their incorrect answers, often backing them up with plausible-sounding but ultimately flawed reasoning. This phenomenon is alarming. It suggests that LLMs can mislead users with confidence, presenting false information as truth.
The study highlights a crucial need for reevaluation. Current testing methods may not adequately capture the reasoning deficiencies of LLMs. The researchers advocate for standardized tests that can effectively identify these weaknesses. Without such measures, the promise of LLMs may be built on shaky ground.
The contrast between Qwen2-Math's advancements and the study's findings is stark. On one hand, we have a tool that enhances mathematical problem-solving. On the other, we face a reality where LLMs struggle with fundamental reasoning. This duality presents a challenge for developers and users alike.
As we embrace the potential of AI, we must also remain vigilant. The allure of LLMs can cloud our judgment. They are not infallible. The hype surrounding their capabilities should be tempered with a healthy dose of skepticism. Users must be aware of the limitations and exercise caution when relying on AI for critical tasks.
Moreover, the implications extend beyond individual users. Businesses and educational institutions must consider the reliability of these models. If LLMs cannot perform basic reasoning, how can they be trusted in high-stakes environments? The consequences of erroneous outputs can be significant, leading to misguided decisions and flawed conclusions.
The journey of AI is a winding road. Innovations like Qwen2-Math represent the bright future of technology. Yet, the shadows cast by studies revealing the limitations of LLMs remind us of the challenges ahead. As we forge ahead, a balanced perspective is essential. We must celebrate advancements while acknowledging shortcomings.
In conclusion, the landscape of AI is both promising and perilous. Qwen2-Math showcases the potential of LLMs to transform mathematical problem-solving. However, the recent study serves as a cautionary tale. It underscores the need for rigorous evaluation and transparency in AI development. As we navigate this complex terrain, we must remain committed to understanding the true capabilities of language models. Only then can we harness their power responsibly and effectively. The future of AI is bright, but it requires careful stewardship.
Alibaba Cloud has unveiled Qwen2-Math, a series of open-source LLMs designed to tackle mathematical problems. This initiative aims to bridge the gap between complex computations and user accessibility. The flagship model, Qwen2-Math-72B-Instruct, boasts an impressive accuracy rate of 84% on the MATH benchmark, which features 12,500 challenging math problems. This performance outshines competitors like OpenAI and Google, marking a significant leap in AI capabilities.
But the story doesn't end there. Qwen2-Math isn't just about numbers. It opens doors for startups, businesses, and academic institutions. The flexible licensing options make it an attractive tool for various applications. Even the smaller model, Qwen2-Math-1.5B, delivers results that rival larger counterparts. This suggests that size isn't everything; efficiency and training matter too.
The implications are vast. With plans to support multiple languages and enhance problem-solving algorithms, Qwen2-Math could democratize access to advanced mathematical tools. Imagine students in remote areas solving complex equations with the help of AI. The potential for educational reform is immense.
However, the excitement surrounding Qwen2-Math is tempered by a sobering reality. A recent study has exposed significant flaws in the logical reasoning capabilities of LLMs. Researchers found that even the most advanced models struggle with basic logical tasks. The study, using a simple problem involving Alice and her siblings, revealed that LLMs often fail to provide correct answers. In fact, more than half of the responses were incorrect.
This raises critical questions about the reliability of LLMs. If these models falter at elementary tasks, what does that mean for their application in more complex scenarios? The researchers noted that LLMs displayed overconfidence in their incorrect answers, often backing them up with plausible-sounding but ultimately flawed reasoning. This phenomenon is alarming. It suggests that LLMs can mislead users with confidence, presenting false information as truth.
The study highlights a crucial need for reevaluation. Current testing methods may not adequately capture the reasoning deficiencies of LLMs. The researchers advocate for standardized tests that can effectively identify these weaknesses. Without such measures, the promise of LLMs may be built on shaky ground.
The contrast between Qwen2-Math's advancements and the study's findings is stark. On one hand, we have a tool that enhances mathematical problem-solving. On the other, we face a reality where LLMs struggle with fundamental reasoning. This duality presents a challenge for developers and users alike.
As we embrace the potential of AI, we must also remain vigilant. The allure of LLMs can cloud our judgment. They are not infallible. The hype surrounding their capabilities should be tempered with a healthy dose of skepticism. Users must be aware of the limitations and exercise caution when relying on AI for critical tasks.
Moreover, the implications extend beyond individual users. Businesses and educational institutions must consider the reliability of these models. If LLMs cannot perform basic reasoning, how can they be trusted in high-stakes environments? The consequences of erroneous outputs can be significant, leading to misguided decisions and flawed conclusions.
The journey of AI is a winding road. Innovations like Qwen2-Math represent the bright future of technology. Yet, the shadows cast by studies revealing the limitations of LLMs remind us of the challenges ahead. As we forge ahead, a balanced perspective is essential. We must celebrate advancements while acknowledging shortcomings.
In conclusion, the landscape of AI is both promising and perilous. Qwen2-Math showcases the potential of LLMs to transform mathematical problem-solving. However, the recent study serves as a cautionary tale. It underscores the need for rigorous evaluation and transparency in AI development. As we navigate this complex terrain, we must remain committed to understanding the true capabilities of language models. Only then can we harness their power responsibly and effectively. The future of AI is bright, but it requires careful stewardship.