Navigating the Future of Video Content Search with AI
July 29, 2024, 3:47 am
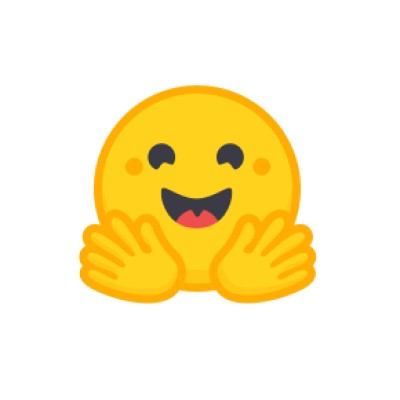
Location: Australia, New South Wales, Concord
Employees: 51-200
Founded date: 2016
Total raised: $494M
In the vast ocean of digital content, finding the right video can feel like searching for a needle in a haystack. The explosion of short video platforms has transformed how we consume media. By 2026, the market for short videos is projected to reach a staggering $292 billion. As the demand for engaging content surges, creators face a daunting challenge: how to quickly locate relevant videos for their marketing needs. Enter the innovative solution from the team at нейро-сети.рф, a service designed to streamline video content search using artificial intelligence.
This article delves into the intricacies of developing a text-based video search service, exploring the challenges, solutions, and technologies that underpin this cutting-edge tool. The goal is to demystify the technical processes involved, making them accessible to both tech-savvy professionals and those new to the field.
**The Problem: A Growing Need for Efficient Video Search**
The landscape of short video content is evolving rapidly. Platforms like TikTok have seen a 95% year-over-year increase in sponsored posts, while traditional platforms like YouTube and Instagram are witnessing declines. This shift creates a pressing need for content creators to find relevant videos quickly. Without efficient tools, the process can be time-consuming and frustrating.
The service developed by нейро-сети.рф aims to bridge this gap. By allowing users to input text queries, the service retrieves videos that match the specified criteria. This capability not only saves time but also enhances the quality of content creation and marketing strategies.
**Exploring Alternative Solutions**
While several services attempt to address the same issue, such as ZebraCat and OpusClip, the approach taken by нейро-сети.рф stands out. The team emphasizes a combination of speed and quality, leveraging advanced AI technologies to optimize the search process.
**The Technical Framework: Building the Service**
At the heart of the service lies the Text2Video Retrieval system. Users input a simple text query, such as "a dog walking on the beach," and the AI works its magic to find relevant video content. This process hinges on several key components:
1. **Data Storage**: Initially, the team utilized MongoDB for data storage, later transitioning to Amazon S3 for scalability. This shift allowed for efficient data management and retrieval.
2. **Data Collection**: The team employed a hybrid approach to gather video content. They sourced videos from platforms like YouTube, ensuring a rich database for the search engine. This method balances speed and quality, allowing for quick retrieval while maintaining high standards.
3. **Data Preprocessing**: Videos often need to be segmented into shorter clips. Using libraries like pyscenedetect, the team extracts scenes from longer videos, ensuring that users receive concise, relevant content.
4. **AI Algorithms**: The core of the service is its AI algorithms. The Vision Language Model generates textual descriptions of video frames, while the Approximate Nearest Neighbor (ANN) algorithm efficiently matches user queries with video content. This dual approach enhances the accuracy and relevance of search results.
5. **Encoder Functionality**: To facilitate the AI's understanding of text, an encoder transforms user queries into numerical vectors. This process allows the AI to compare and match queries with video descriptions effectively.
**Training the AI: A Continuous Learning Process**
Training the AI is akin to nurturing a young plant. It requires care, attention, and the right environment to thrive. The team employed contrastive learning techniques to refine the AI's ability to distinguish between relevant and irrelevant content. By training the model on specific datasets, they ensured that it could accurately interpret user queries and deliver precise results.
**Measuring Success: Precision Metrics**
To gauge the effectiveness of the AI, the team implemented metrics like Precision@k. This metric evaluates how well the model retrieves relevant videos among the top k results. A higher precision score indicates a more effective search, enhancing user satisfaction.
**The Future of Video Search: A Promising Horizon**
As the digital landscape continues to evolve, the need for efficient video search solutions will only grow. The service developed by нейро-сети.рф represents a significant step forward in addressing this challenge. By harnessing the power of AI, the team has created a tool that not only simplifies the search process but also empowers content creators to engage their audiences more effectively.
In conclusion, the journey of developing a text-based video search service is a testament to the potential of AI in transforming how we interact with digital content. As technology advances, we can expect even more innovative solutions to emerge, making the search for relevant video content as seamless as a gentle wave lapping at the shore. The future is bright, and the possibilities are endless.
This article delves into the intricacies of developing a text-based video search service, exploring the challenges, solutions, and technologies that underpin this cutting-edge tool. The goal is to demystify the technical processes involved, making them accessible to both tech-savvy professionals and those new to the field.
**The Problem: A Growing Need for Efficient Video Search**
The landscape of short video content is evolving rapidly. Platforms like TikTok have seen a 95% year-over-year increase in sponsored posts, while traditional platforms like YouTube and Instagram are witnessing declines. This shift creates a pressing need for content creators to find relevant videos quickly. Without efficient tools, the process can be time-consuming and frustrating.
The service developed by нейро-сети.рф aims to bridge this gap. By allowing users to input text queries, the service retrieves videos that match the specified criteria. This capability not only saves time but also enhances the quality of content creation and marketing strategies.
**Exploring Alternative Solutions**
While several services attempt to address the same issue, such as ZebraCat and OpusClip, the approach taken by нейро-сети.рф stands out. The team emphasizes a combination of speed and quality, leveraging advanced AI technologies to optimize the search process.
**The Technical Framework: Building the Service**
At the heart of the service lies the Text2Video Retrieval system. Users input a simple text query, such as "a dog walking on the beach," and the AI works its magic to find relevant video content. This process hinges on several key components:
1. **Data Storage**: Initially, the team utilized MongoDB for data storage, later transitioning to Amazon S3 for scalability. This shift allowed for efficient data management and retrieval.
2. **Data Collection**: The team employed a hybrid approach to gather video content. They sourced videos from platforms like YouTube, ensuring a rich database for the search engine. This method balances speed and quality, allowing for quick retrieval while maintaining high standards.
3. **Data Preprocessing**: Videos often need to be segmented into shorter clips. Using libraries like pyscenedetect, the team extracts scenes from longer videos, ensuring that users receive concise, relevant content.
4. **AI Algorithms**: The core of the service is its AI algorithms. The Vision Language Model generates textual descriptions of video frames, while the Approximate Nearest Neighbor (ANN) algorithm efficiently matches user queries with video content. This dual approach enhances the accuracy and relevance of search results.
5. **Encoder Functionality**: To facilitate the AI's understanding of text, an encoder transforms user queries into numerical vectors. This process allows the AI to compare and match queries with video descriptions effectively.
**Training the AI: A Continuous Learning Process**
Training the AI is akin to nurturing a young plant. It requires care, attention, and the right environment to thrive. The team employed contrastive learning techniques to refine the AI's ability to distinguish between relevant and irrelevant content. By training the model on specific datasets, they ensured that it could accurately interpret user queries and deliver precise results.
**Measuring Success: Precision Metrics**
To gauge the effectiveness of the AI, the team implemented metrics like Precision@k. This metric evaluates how well the model retrieves relevant videos among the top k results. A higher precision score indicates a more effective search, enhancing user satisfaction.
**The Future of Video Search: A Promising Horizon**
As the digital landscape continues to evolve, the need for efficient video search solutions will only grow. The service developed by нейро-сети.рф represents a significant step forward in addressing this challenge. By harnessing the power of AI, the team has created a tool that not only simplifies the search process but also empowers content creators to engage their audiences more effectively.
In conclusion, the journey of developing a text-based video search service is a testament to the potential of AI in transforming how we interact with digital content. As technology advances, we can expect even more innovative solutions to emerge, making the search for relevant video content as seamless as a gentle wave lapping at the shore. The future is bright, and the possibilities are endless.