Robots That Think: The Dawn of Embodied Reasoning** **
July 25, 2024, 9:18 pm
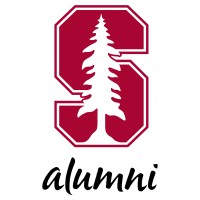
Location: United Kingdom, England, City of London
Employees: 10001+
Founded date: 1885
**
In the world of robotics, the quest for intelligence is relentless. Imagine a robot that doesn’t just follow commands but thinks before it acts. This is the promise of a new technique called Embodied Chain-of-Thought Reasoning (ECoT). Researchers from prestigious institutions like UC Berkeley and Stanford are pioneering this approach, aiming to enhance how robots perceive and interact with their environment.
Traditionally, robots have been like obedient dogs, responding to commands without understanding the context. They execute tasks but often stumble when faced with unexpected situations. Enter ECoT, a method that empowers robots to reason about their actions. It’s akin to teaching a child not just to follow instructions but to understand the “why” behind them.
At the heart of ECoT is the concept of vision-language-action models (VLAs). These models integrate visual inputs with language instructions, allowing robots to interpret their surroundings and respond appropriately. Think of it as giving robots a pair of glasses that help them see the world more clearly, combined with a brain that can process complex instructions.
The challenge lies in the inherent limitations of current VLAs. While they excel at mapping observations to actions, they lack the reasoning capabilities that large language models (LLMs) possess. LLMs can break down complex problems into manageable steps, a skill that VLAs need to master. The researchers believe that by training VLAs to think textually about their plans and environments, they can significantly improve robot performance.
However, this is no simple task. VLAs operate on smaller, less sophisticated models compared to their LLM counterparts. They must not only understand tasks but also the nuances of their environment and their own state. It’s not enough to think carefully; they must also look carefully. This dual focus is what ECoT aims to achieve.
ECoT combines high-level semantic reasoning with grounded, embodied reasoning. It’s like teaching a robot to not only plan a route but also to navigate obstacles in real-time. The researchers have developed a pipeline to generate synthetic training data, allowing VLAs to learn from their surroundings. This involves using pre-trained object detectors and language models to annotate existing datasets, creating a rich learning environment for the robots.
The results are promising. In tests using OpenVLA, a model built on Llama-2, ECoT improved task success rates by 28%. This leap in performance came without the need for additional training data, a significant advantage in the resource-intensive field of robotics. Moreover, ECoT provides clarity in understanding failures. When a robot makes a mistake, the reasoning steps are expressed in natural language, making it easier to trace back and identify the error.
This transparency is crucial. It allows human operators to interact with the robot’s decision-making process, offering corrections through natural language. Instead of relying on complex teleoperation systems, users can simply adjust the robot’s reasoning chains. This shift could revolutionize how humans and robots collaborate.
ECoT is part of a broader movement to integrate foundation models into robotic systems. These models, trained on vast amounts of data, can fill gaps in current robotics technology. They are being utilized across various aspects of robotics, from planning actions to understanding environments. As the industry evolves, the potential for foundation models optimized for robotics is immense.
The implications of ECoT extend beyond improved performance. They touch on the very nature of human-robot interaction. As robots become more capable of reasoning, they will be better equipped to assist in complex tasks, from industrial automation to personal assistance. Imagine a robot that not only understands your commands but can also anticipate your needs, adjusting its actions accordingly.
However, the journey is just beginning. The integration of ECoT into practical applications will require further research and development. The challenges of real-world environments, where unpredictability reigns, must be addressed. Yet, the potential rewards are vast. A future where robots can think and reason like humans could transform industries and daily life.
In conclusion, the development of Embodied Chain-of-Thought Reasoning marks a significant milestone in robotics. It’s a step toward creating machines that don’t just execute commands but understand their actions. As researchers continue to refine this technology, we stand on the brink of a new era in robotics—one where machines are not just tools but intelligent partners in our endeavors. The road ahead is filled with challenges, but the promise of smarter, more capable robots is a vision worth pursuing.
In the world of robotics, the quest for intelligence is relentless. Imagine a robot that doesn’t just follow commands but thinks before it acts. This is the promise of a new technique called Embodied Chain-of-Thought Reasoning (ECoT). Researchers from prestigious institutions like UC Berkeley and Stanford are pioneering this approach, aiming to enhance how robots perceive and interact with their environment.
Traditionally, robots have been like obedient dogs, responding to commands without understanding the context. They execute tasks but often stumble when faced with unexpected situations. Enter ECoT, a method that empowers robots to reason about their actions. It’s akin to teaching a child not just to follow instructions but to understand the “why” behind them.
At the heart of ECoT is the concept of vision-language-action models (VLAs). These models integrate visual inputs with language instructions, allowing robots to interpret their surroundings and respond appropriately. Think of it as giving robots a pair of glasses that help them see the world more clearly, combined with a brain that can process complex instructions.
The challenge lies in the inherent limitations of current VLAs. While they excel at mapping observations to actions, they lack the reasoning capabilities that large language models (LLMs) possess. LLMs can break down complex problems into manageable steps, a skill that VLAs need to master. The researchers believe that by training VLAs to think textually about their plans and environments, they can significantly improve robot performance.
However, this is no simple task. VLAs operate on smaller, less sophisticated models compared to their LLM counterparts. They must not only understand tasks but also the nuances of their environment and their own state. It’s not enough to think carefully; they must also look carefully. This dual focus is what ECoT aims to achieve.
ECoT combines high-level semantic reasoning with grounded, embodied reasoning. It’s like teaching a robot to not only plan a route but also to navigate obstacles in real-time. The researchers have developed a pipeline to generate synthetic training data, allowing VLAs to learn from their surroundings. This involves using pre-trained object detectors and language models to annotate existing datasets, creating a rich learning environment for the robots.
The results are promising. In tests using OpenVLA, a model built on Llama-2, ECoT improved task success rates by 28%. This leap in performance came without the need for additional training data, a significant advantage in the resource-intensive field of robotics. Moreover, ECoT provides clarity in understanding failures. When a robot makes a mistake, the reasoning steps are expressed in natural language, making it easier to trace back and identify the error.
This transparency is crucial. It allows human operators to interact with the robot’s decision-making process, offering corrections through natural language. Instead of relying on complex teleoperation systems, users can simply adjust the robot’s reasoning chains. This shift could revolutionize how humans and robots collaborate.
ECoT is part of a broader movement to integrate foundation models into robotic systems. These models, trained on vast amounts of data, can fill gaps in current robotics technology. They are being utilized across various aspects of robotics, from planning actions to understanding environments. As the industry evolves, the potential for foundation models optimized for robotics is immense.
The implications of ECoT extend beyond improved performance. They touch on the very nature of human-robot interaction. As robots become more capable of reasoning, they will be better equipped to assist in complex tasks, from industrial automation to personal assistance. Imagine a robot that not only understands your commands but can also anticipate your needs, adjusting its actions accordingly.
However, the journey is just beginning. The integration of ECoT into practical applications will require further research and development. The challenges of real-world environments, where unpredictability reigns, must be addressed. Yet, the potential rewards are vast. A future where robots can think and reason like humans could transform industries and daily life.
In conclusion, the development of Embodied Chain-of-Thought Reasoning marks a significant milestone in robotics. It’s a step toward creating machines that don’t just execute commands but understand their actions. As researchers continue to refine this technology, we stand on the brink of a new era in robotics—one where machines are not just tools but intelligent partners in our endeavors. The road ahead is filled with challenges, but the promise of smarter, more capable robots is a vision worth pursuing.