The Rising Costs of Artificial Intelligence: A Deep Dive into the Financial Implications
July 3, 2024, 3:43 am
Google
Location: United States, New York
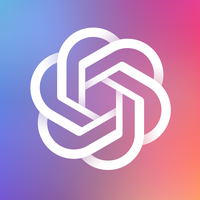
Location: United States, California, San Francisco
Employees: 201-500
Founded date: 2015
Total raised: $11.57B
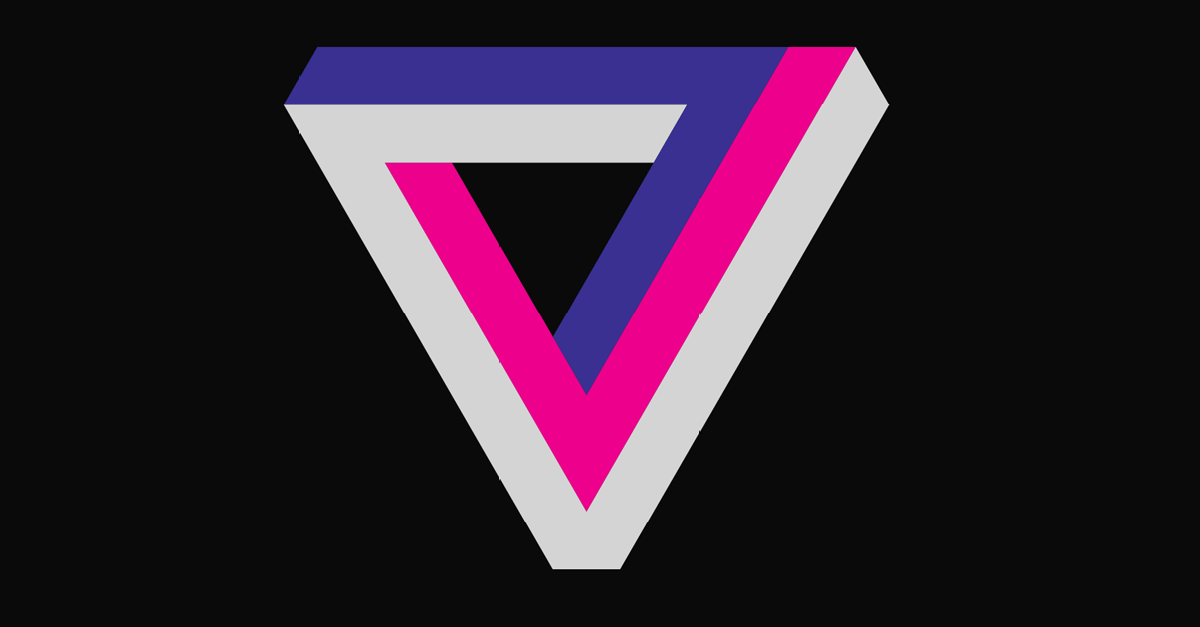
Location: United States, New York
Employees: 51-200
Founded date: 2011
Total raised: $400K
In today's rapidly evolving technological landscape, artificial intelligence (AI) is experiencing explosive growth. The pace of AI integration is accelerating worldwide, permeating diverse industries such as healthcare, education, finance, media, and marketing. The competitive advantage of AI in automating processes and personalizing services is compelling companies to swiftly incorporate these technologies into their business operations.
According to forecasts, the global AI market is projected to reach $184 billion in 2024, a 35% increase from 2023. With an annual growth rate of 28.46%, the market is expected to surpass $826 billion by 2030. However, more optimistic estimates suggest an annual growth rate of 35.7% from 2024 to 2030, with the market size exceeding $1.339 trillion by 2030.
Yet, as technology advances, so do the associated costs. Two key factors contribute to the escalating expenses: the increasing scale and cost of developing AI models, and the growing global demand for AI services necessitating more data centers and infrastructure to support them.
Epoch AI, an AI research institute, reports that 47-67% of AI expenditures are allocated to AI chips, server components, and peripheral equipment. Research and development personnel costs account for 29-49%, while electricity consumption represents the remaining 2-6% of expenses.
Let's delve deeper into the breakdown of AI costs and attempt to assess the magnitude of these expenditures.
Large Language Models (LLM)
Leading AI applications today, such as ChatGPT, are built on large language models (LLMs) – systems that process vast amounts of data, including books, articles, online comments, and videos, to provide optimal responses to user queries. Major developers are betting on making large language models even larger as the path to more advanced AI – potentially surpassing human capabilities – involves scaling up these models.
In April 2023, OpenAI's CEO, Sam Altman, stated, "I think we've reached the end of the era where giant models are emerging. We'll make them better in other ways." However, the focus remains on increasing computational power, data volume, and the exponential growth in training costs.
According to Stanford University, advanced AI models are becoming increasingly expensive, with the costs of training modern AI models reaching unprecedented levels. For instance, the training of OpenAI's GPT-4 utilized computational resources costing around $78 million (some sources cite over $100 million), while Google's Gemini Ultra incurred costs of $191 million.
Epoch AI's research indicates that the computational power of computers used to train the latest AI models is increasing by 4-5 times annually. If the trend of rising costs continues, the cost of training advanced AI models is projected to exceed $1 billion by 2027.
In an interview with CNBC in early April, Dario Amodei, CEO of AI startup Anthropic, predicted that training model costs would reach around $1 billion next year. By 2025 or 2026, these costs are expected to rise to $5-10 billion, with a potential to exceed $100 billion.
Chips
In 2023, the AI chip market reached $53.6 billion. Forecasts suggest that by the end of 2024, this figure will increase to $71.3 billion, as the excitement around AI applications continues to grow. Long-term projections indicate that the market will exceed $90 billion in 2025.
The rapid growth of artificial intelligence is accompanied by a chip shortage, directly impacting their costs. Nvidia, the market leader, with 60-70% of global AI chip shipments, recently became the world's largest company with a market capitalization exceeding $3.3 trillion, surpassing Microsoft and Apple.
According to analysts at Raymond James, Nvidia currently sells H100 graphics processors for $25,000 to $30,000 each, while the next-generation graphics processor for AI, Blackwell, will cost $30,000 to $40,000. The number of graphics processors required for AI training is already in the millions.
Industry leaders Microsoft and OpenAI plan to create a unique supercomputer called "Stargate" for AI research, utilizing millions of specialized server chips. It will be the centerpiece of a five-stage plan to build a series of supercomputers over the next six years, with the launch of "Stargate" scheduled for 2028.
Data Centers
The advancement of artificial intelligence is increasing the demand for computational power, data storage, and cloud services, driving a significant push for the modernization and construction of new data centers. Currently, there are approximately 11,000 data centers operating worldwide, with the United States leading with 5,381 centers, followed by Germany with 521 and the United Kingdom with 514. Russia ranks 9th with 297 data centers.
In the U.S., the absolute leader in the number of data centers, 46% more centers were built in 2023 than in 2022.
In August 2023, Nvidia's CEO, Jensen Huang, forecasted that $1 trillion would be spent on AI data center modernization within four years. The cost of data center operations amounts to around $1 trillion, representing a quarter of a trillion dollars in annual capital expenditures. The bulk of these expenses will likely fall on leading cloud providers – hyper-scalers like Amazon, Microsoft, and Google, joined by Meta and other major tech companies.
Researchers at Dell'Oro Group estimate that companies will spend $294 billion on data center construction and equipment in 2024, compared to $193 billion in 2020. These expenditures include the transition to GPU-accelerated servers – servers equipped with graphics processors and custom accelerators. In the first quarter of 2024, GPU-accelerated servers accounted for over half of all server sales. The GPU accelerated server market is expected to grow by over 80% in 2024.
Aside from constructing new data centers, the density of racks with hard drives, processors, and cooling systems in existing centers will increase, with an aggregate annual growth rate of 7.8%. By 2027, the average rack power is expected to reach 50 kW, surpassing the current average of 36 kW.
Electricity
Processing a request in ChatGPT requires nearly 10 times more electricity than a Google search. This disparity is one of the primary reasons for the projected increase in global energy consumption in the near future. Neural networks training on internet data have an insatiable appetite for electricity, as do the cooling systems required to prevent overheating.
Existing data centers collectively have the capacity to consume 508 terawatt-hours of electricity annually if they were to operate continuously. This exceeds the total annual electricity production of Italy or Australia. By 2034, global data center energy consumption is expected to surpass 1,580 terawatt-hours, roughly equivalent to India's total energy consumption.
According to Goldman Sachs Research, by 2030:
- The demand for electricity for data centers will increase by 160%.
- Data center electricity consumption will rise from 1-2% of global energy to 3-4%.
- In the U.S., which accounts for 49% of data centers, the increase will be even greater – from 3% to 8%.
- Around 20% of total data center energy consumption will be attributed to artificial intelligence.
Such a surge in energy consumption necessitates unconventional approaches to address the energy generation challenge.
In May 2023, Microsoft announced an agreement to purchase electricity from Helion Energy, a company planning to start generating nuclear energy through fusion by 2028 (several working prototypes have already been built). Microsoft later posted a job listing for a nuclear technology program manager tasked with developing a strategy "to power the data centers housing Microsoft Cloud and artificial intelligence." The company is expected to focus on using microreactors and small modular reactors, which are much cheaper to manufacture and operate than large nuclear reactors.
Talent
The battle for AI talent has never been more fierce: every company in the field is vying for a very small pool of researchers and engineers. Today, the compensation of AI specialists is a battleground. Recognizing that sought-after AI professionals may receive multiple job offers, many companies are offering salaries in the six-figure range, along with bonuses and stock grants, to attract more experienced employees.
Company leaders face the challenge of not only attracting highly qualified employees but also retaining them from moving to competitors.
For example, it is widely known that when Google co-founder Sergey Brin personally called an employee who was considering leaving for OpenAI. Brin's phone call, along with other promises and additional compensation, convinced the employee to stay at Google.
Forecasts indicate that from 2022 to 2032, the number of professionals engaged in computer and information research will increase by 23%, significantly higher than the average across all professions.
According to Glassdoor, the average annual salary for a machine learning engineer ranges from $131,000 to $210,000. The most common salary range for engineering positions listed on the OpenAI website is $200,000 to $370,000, with vacancies for more qualified specialists ranging from $300,000 to $450,000. In addition to salary, there are also bonuses referred to as "Profit Participation Units" (PPUs). For example, with a $300,000 salary, PPUs could amount to $500,000 per year.
The implementation of artificial intelligence has led to an unprecedented demand for specialists in various industries. Companies like Netflix or Walmart are actively seeking employees to develop innovative solutions, improve customer service quality, optimize supply chains, and make AI-based decisions. In 2023, a job posting for a product manager for the machine learning platform at Netflix offered candidates a salary plus bonuses of up to $900,000 per year, sparking excitement on social media.
However, salary alone does not solve everything. Aravind Srinivas, CEO of AI startup Perplexity, shared the challenges of hiring a highly qualified generative AI specialist: "I tried to hire a senior researcher from Meta, and you know what he said? 'Come to me when you have 10,000 Nvidia H100 graphics processors.'"
Legal Expenses
When discussing the cost of artificial intelligence, one cannot overlook legal expenses. It is no secret that AI training utilizes all available information – news, literary works, bulletin board messages, Wikipedia articles, computer programs, photos, podcasts, and videos. Often, copyright laws are violated.
Currently, there are numerous lawsuits against AI startups and their investors for copyright infringement. Plaintiffs include leading news portals like the New York Times, as well as individual writers, book authors, artists, and photographers who believe their works were used to generate profits without compensation.
While the lawsuits do not specify the exact monetary demands, they refer to "billions of dollars in established and actual damages" related to "unlawful copying and use of unique texts."
New lawsuits are continuously being filed. This week, the world's largest record companies – Sony Music, Universal Music Group, and Warner Records – filed lawsuits against two AI startups, Suno and Audio, for alleged copyright infringement. They are seeking compensation of $150,000 for each work used.
To avoid losing in court, AI startups are forced to expand their legal departments, hiring top lawyers from BigTech companies and engaging large law firms, whose services come at a high cost. The question of whether they will win in court remains open.
According to forecasts, the global AI market is projected to reach $184 billion in 2024, a 35% increase from 2023. With an annual growth rate of 28.46%, the market is expected to surpass $826 billion by 2030. However, more optimistic estimates suggest an annual growth rate of 35.7% from 2024 to 2030, with the market size exceeding $1.339 trillion by 2030.
Yet, as technology advances, so do the associated costs. Two key factors contribute to the escalating expenses: the increasing scale and cost of developing AI models, and the growing global demand for AI services necessitating more data centers and infrastructure to support them.
Epoch AI, an AI research institute, reports that 47-67% of AI expenditures are allocated to AI chips, server components, and peripheral equipment. Research and development personnel costs account for 29-49%, while electricity consumption represents the remaining 2-6% of expenses.
Let's delve deeper into the breakdown of AI costs and attempt to assess the magnitude of these expenditures.
Large Language Models (LLM)
Leading AI applications today, such as ChatGPT, are built on large language models (LLMs) – systems that process vast amounts of data, including books, articles, online comments, and videos, to provide optimal responses to user queries. Major developers are betting on making large language models even larger as the path to more advanced AI – potentially surpassing human capabilities – involves scaling up these models.
In April 2023, OpenAI's CEO, Sam Altman, stated, "I think we've reached the end of the era where giant models are emerging. We'll make them better in other ways." However, the focus remains on increasing computational power, data volume, and the exponential growth in training costs.
According to Stanford University, advanced AI models are becoming increasingly expensive, with the costs of training modern AI models reaching unprecedented levels. For instance, the training of OpenAI's GPT-4 utilized computational resources costing around $78 million (some sources cite over $100 million), while Google's Gemini Ultra incurred costs of $191 million.
Epoch AI's research indicates that the computational power of computers used to train the latest AI models is increasing by 4-5 times annually. If the trend of rising costs continues, the cost of training advanced AI models is projected to exceed $1 billion by 2027.
In an interview with CNBC in early April, Dario Amodei, CEO of AI startup Anthropic, predicted that training model costs would reach around $1 billion next year. By 2025 or 2026, these costs are expected to rise to $5-10 billion, with a potential to exceed $100 billion.
Chips
In 2023, the AI chip market reached $53.6 billion. Forecasts suggest that by the end of 2024, this figure will increase to $71.3 billion, as the excitement around AI applications continues to grow. Long-term projections indicate that the market will exceed $90 billion in 2025.
The rapid growth of artificial intelligence is accompanied by a chip shortage, directly impacting their costs. Nvidia, the market leader, with 60-70% of global AI chip shipments, recently became the world's largest company with a market capitalization exceeding $3.3 trillion, surpassing Microsoft and Apple.
According to analysts at Raymond James, Nvidia currently sells H100 graphics processors for $25,000 to $30,000 each, while the next-generation graphics processor for AI, Blackwell, will cost $30,000 to $40,000. The number of graphics processors required for AI training is already in the millions.
Industry leaders Microsoft and OpenAI plan to create a unique supercomputer called "Stargate" for AI research, utilizing millions of specialized server chips. It will be the centerpiece of a five-stage plan to build a series of supercomputers over the next six years, with the launch of "Stargate" scheduled for 2028.
Data Centers
The advancement of artificial intelligence is increasing the demand for computational power, data storage, and cloud services, driving a significant push for the modernization and construction of new data centers. Currently, there are approximately 11,000 data centers operating worldwide, with the United States leading with 5,381 centers, followed by Germany with 521 and the United Kingdom with 514. Russia ranks 9th with 297 data centers.
In the U.S., the absolute leader in the number of data centers, 46% more centers were built in 2023 than in 2022.
In August 2023, Nvidia's CEO, Jensen Huang, forecasted that $1 trillion would be spent on AI data center modernization within four years. The cost of data center operations amounts to around $1 trillion, representing a quarter of a trillion dollars in annual capital expenditures. The bulk of these expenses will likely fall on leading cloud providers – hyper-scalers like Amazon, Microsoft, and Google, joined by Meta and other major tech companies.
Researchers at Dell'Oro Group estimate that companies will spend $294 billion on data center construction and equipment in 2024, compared to $193 billion in 2020. These expenditures include the transition to GPU-accelerated servers – servers equipped with graphics processors and custom accelerators. In the first quarter of 2024, GPU-accelerated servers accounted for over half of all server sales. The GPU accelerated server market is expected to grow by over 80% in 2024.
Aside from constructing new data centers, the density of racks with hard drives, processors, and cooling systems in existing centers will increase, with an aggregate annual growth rate of 7.8%. By 2027, the average rack power is expected to reach 50 kW, surpassing the current average of 36 kW.
Electricity
Processing a request in ChatGPT requires nearly 10 times more electricity than a Google search. This disparity is one of the primary reasons for the projected increase in global energy consumption in the near future. Neural networks training on internet data have an insatiable appetite for electricity, as do the cooling systems required to prevent overheating.
Existing data centers collectively have the capacity to consume 508 terawatt-hours of electricity annually if they were to operate continuously. This exceeds the total annual electricity production of Italy or Australia. By 2034, global data center energy consumption is expected to surpass 1,580 terawatt-hours, roughly equivalent to India's total energy consumption.
According to Goldman Sachs Research, by 2030:
- The demand for electricity for data centers will increase by 160%.
- Data center electricity consumption will rise from 1-2% of global energy to 3-4%.
- In the U.S., which accounts for 49% of data centers, the increase will be even greater – from 3% to 8%.
- Around 20% of total data center energy consumption will be attributed to artificial intelligence.
Such a surge in energy consumption necessitates unconventional approaches to address the energy generation challenge.
In May 2023, Microsoft announced an agreement to purchase electricity from Helion Energy, a company planning to start generating nuclear energy through fusion by 2028 (several working prototypes have already been built). Microsoft later posted a job listing for a nuclear technology program manager tasked with developing a strategy "to power the data centers housing Microsoft Cloud and artificial intelligence." The company is expected to focus on using microreactors and small modular reactors, which are much cheaper to manufacture and operate than large nuclear reactors.
Talent
The battle for AI talent has never been more fierce: every company in the field is vying for a very small pool of researchers and engineers. Today, the compensation of AI specialists is a battleground. Recognizing that sought-after AI professionals may receive multiple job offers, many companies are offering salaries in the six-figure range, along with bonuses and stock grants, to attract more experienced employees.
Company leaders face the challenge of not only attracting highly qualified employees but also retaining them from moving to competitors.
For example, it is widely known that when Google co-founder Sergey Brin personally called an employee who was considering leaving for OpenAI. Brin's phone call, along with other promises and additional compensation, convinced the employee to stay at Google.
Forecasts indicate that from 2022 to 2032, the number of professionals engaged in computer and information research will increase by 23%, significantly higher than the average across all professions.
According to Glassdoor, the average annual salary for a machine learning engineer ranges from $131,000 to $210,000. The most common salary range for engineering positions listed on the OpenAI website is $200,000 to $370,000, with vacancies for more qualified specialists ranging from $300,000 to $450,000. In addition to salary, there are also bonuses referred to as "Profit Participation Units" (PPUs). For example, with a $300,000 salary, PPUs could amount to $500,000 per year.
The implementation of artificial intelligence has led to an unprecedented demand for specialists in various industries. Companies like Netflix or Walmart are actively seeking employees to develop innovative solutions, improve customer service quality, optimize supply chains, and make AI-based decisions. In 2023, a job posting for a product manager for the machine learning platform at Netflix offered candidates a salary plus bonuses of up to $900,000 per year, sparking excitement on social media.
However, salary alone does not solve everything. Aravind Srinivas, CEO of AI startup Perplexity, shared the challenges of hiring a highly qualified generative AI specialist: "I tried to hire a senior researcher from Meta, and you know what he said? 'Come to me when you have 10,000 Nvidia H100 graphics processors.'"
Legal Expenses
When discussing the cost of artificial intelligence, one cannot overlook legal expenses. It is no secret that AI training utilizes all available information – news, literary works, bulletin board messages, Wikipedia articles, computer programs, photos, podcasts, and videos. Often, copyright laws are violated.
Currently, there are numerous lawsuits against AI startups and their investors for copyright infringement. Plaintiffs include leading news portals like the New York Times, as well as individual writers, book authors, artists, and photographers who believe their works were used to generate profits without compensation.
While the lawsuits do not specify the exact monetary demands, they refer to "billions of dollars in established and actual damages" related to "unlawful copying and use of unique texts."
New lawsuits are continuously being filed. This week, the world's largest record companies – Sony Music, Universal Music Group, and Warner Records – filed lawsuits against two AI startups, Suno and Audio, for alleged copyright infringement. They are seeking compensation of $150,000 for each work used.
To avoid losing in court, AI startups are forced to expand their legal departments, hiring top lawyers from BigTech companies and engaging large law firms, whose services come at a high cost. The question of whether they will win in court remains open.