The Evolution of Data Practitioners in the AI Era
June 11, 2024, 9:58 pm
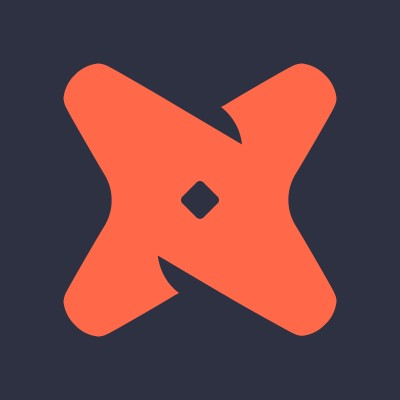
Location: United States, Pennsylvania, Philadelphia
Employees: 51-200
Founded date: 2016
Total raised: $372M
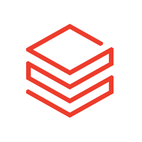
Location: Netherlands, North Holland, Amsterdam
Employees: 1001-5000
Founded date: 2013
Total raised: $4.31B
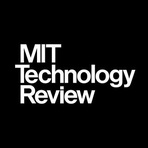
Location: United States, Massachusetts, Cambridge
Employees: 501-1000
Founded date: 1899
Data professionals are at the forefront of shaping AI applications and improving data quality for organizations to stay competitive in the evolving landscape.
In a recent report by dbt Labs and Databricks, the critical role of data practitioners in managing data pipelines and processes was highlighted. The findings emphasized the importance of data teams in supporting business strategy, operations, and enhancing the quality of AI outputs.
Data jobs are rapidly evolving from traditional roles to more integrated, strategic positions within organizations. Data intelligence platforms are being integrated to bridge the gap between technical and business units, enhancing data visibility and transparency.
As organizations invest more in data as the cornerstone of their AI strategy, data practitioners play a central role in ensuring data quality, governance, and the effective deployment of AI technologies. The concept of "Data as a Product" is emerging, requiring practitioners to adopt a product management mindset to prioritize tasks with significant business impact.
Despite the progress, data challenges such as data silos, processing speed, data sufficiency, and monitoring lineage remain obstacles for organizations to achieve their AI aspirations. Companies need a unified approach to query and understand data across silos.
One key technique to improve data quality for AI outputs is retrieval-augmented generation (RAG), which helps mitigate issues like bias and hallucinations. By grounding AI models with verifiable external knowledge sources, organizations can produce higher-quality, specialized, and verifiable AI outputs.
The transformation of data roles, responsibilities, and workflows is evident as AI becomes embedded in how teams work. Analytics engineers, a new breed of data practitioners, are bridging the gap between data and business needs, translating requirements into effective data transformations essential for business strategy and operations.
In conclusion, data practitioners are pivotal in shaping the future of AI applications and data quality for organizations to thrive in the AI era. The evolving landscape requires organizations to adapt and invest in strategies that prioritize data quality, governance, and the effective deployment of AI technologies.
In a recent report by dbt Labs and Databricks, the critical role of data practitioners in managing data pipelines and processes was highlighted. The findings emphasized the importance of data teams in supporting business strategy, operations, and enhancing the quality of AI outputs.
Data jobs are rapidly evolving from traditional roles to more integrated, strategic positions within organizations. Data intelligence platforms are being integrated to bridge the gap between technical and business units, enhancing data visibility and transparency.
As organizations invest more in data as the cornerstone of their AI strategy, data practitioners play a central role in ensuring data quality, governance, and the effective deployment of AI technologies. The concept of "Data as a Product" is emerging, requiring practitioners to adopt a product management mindset to prioritize tasks with significant business impact.
Despite the progress, data challenges such as data silos, processing speed, data sufficiency, and monitoring lineage remain obstacles for organizations to achieve their AI aspirations. Companies need a unified approach to query and understand data across silos.
One key technique to improve data quality for AI outputs is retrieval-augmented generation (RAG), which helps mitigate issues like bias and hallucinations. By grounding AI models with verifiable external knowledge sources, organizations can produce higher-quality, specialized, and verifiable AI outputs.
The transformation of data roles, responsibilities, and workflows is evident as AI becomes embedded in how teams work. Analytics engineers, a new breed of data practitioners, are bridging the gap between data and business needs, translating requirements into effective data transformations essential for business strategy and operations.
In conclusion, data practitioners are pivotal in shaping the future of AI applications and data quality for organizations to thrive in the AI era. The evolving landscape requires organizations to adapt and invest in strategies that prioritize data quality, governance, and the effective deployment of AI technologies.